Deep Facial Diagnosis: Deep Transfer Learning From Face Recognition to Facial Diagnosis
ABSTRACT The relationship between face and disease has been discussed from thousands years ago, which leads to the occurrence of facial diagnosis. The objective here is to explore the possibility of identifying diseases from uncontrolled 2D face images by deep learning techniques. In this paper, we propose using deep transfer learning from face recognition to perform the computer-aided facial diagnosis on various diseases. In the experiments, we perform the computer-aided facial diagnosis on single (beta-thalassemia) and multiple diseases (beta-thalassemia, hyperthyroidism, Down syndrome, and leprosy) with a relatively small dataset. The overall top-1 accuracy by deep transfer learning from face recognition can reach over 90% which outperforms the performance of both traditional machine learning methods and clinicians in the experiments. In practical, collecting disease-specific face images is complex, expensive and time consuming, and imposes ethical limitations due to personal data treatment. Therefore, the datasets of facial diagnosis related researches are private and generally small comparing with the ones of other machine learning application areas. The success of deep transfer learning applications in the facial diagnosis with a small dataset could provide a low-cost and noninvasive way for disease screening and detection.
INDEX TERMS Facial diagnosis, deep transfer learning (DTL), face recognition, beta-thalassemia, hyperthyroidism, down syndrome, leprosy.
- INTRODUCTION
Thousands years ago, Huangdi Neijing [1], the fundamental doctrinal source for Chinese medicine, recorded ‘‘Qi and blood in the twelve Channels and three hundred and sixty-five Collaterals all flow to the face and infuse into the Kongqiao (the seven orifices on the face).’’ It indicates the pathological changes of the internal organs can be reflected in the face of the relevant areas. In China, one experienced doctor can observe the patient’s facial features to know the patient’s whole and local lesions, which is called ‘‘facial diagnosis’’. Similar theories also existed in ancient India and ancient Greece. Nowadays, facial diagnosis refers to that practitioners perform disease diagnosis by observing facial features. The shortcoming of facial diagnosis is that for getting a high accuracy facial diagnosis requires doctors to have a large amount of practical experience. Modern medical researches [11], [12], [30] indicate that, indeed, many diseases will express corresponding specific features on human faces. Nowadays, it is still difficult for people to take a medical examination in many rural and underdeveloped areas because of the limited medical resources, which leads to delays in treatment in many cases. Even in metropolises, limitations including the high cost, long queuing time in hospital and the doctor-patient contradiction which leads to medical disputes still exist. Computer-aided facial diagnosis enables us to carry out non-invasive screening and detection of diseases quickly and easily. Therefore, if facial diagnosis can be proved effective with an acceptable error rate, it will be with great potential. With the help of artificial intelligence, we could explore the relationship between face and disease with a quantitative approach. In recent years, deep learning technology improves the state of the art in many areas for its good performances especially in computer vision. Deep learning inspired by the structure of human brains is to use a multiple-layer structure to perform nonlinear information processing and abstraction for feature learning. It has shown its best performance in ImageNet Large Scale Visual Recognition Challenge (ILSVRC) [42] from 2012. As the challenge progresses, several classic deep neural network models [2]–[6], [36] appeared such as AlexNet, VGGNet, ResNet, Inception-ResNet and SENet. The results of ILSVRCs have fully shown that learning features by deep learning methods can express the inherent information of the data more effectively than the artificial features. Up to now, deep learning has become one of the newest trends in artificial intelligence researches. Face recognition refers to the technology of verifying or identifying the identity of subjects from faces in images or videos. It is a hot topic in the field of computer vision. Face verification is the task of comparing a candidate face to another, and verifying whether it is a match or not. It is a one-to-one mapping. Face identification is the task of matching a given face image to one in a database of faces. It is a one-to-many mapping. These two can be implemented by separate algorithm frameworks, or they can be unified into one framework by metric learning. With the development of deep learning in recent years, traditional face recognition technology has gradually been replaced by deep learning methods. Convolutional Neural Network (CNN) is the most commonly used deep learning method in face recognition. The CNN architectures [7], [8], [27] for face recognition including FaceNet, VGG-Face, DeepFace and ResNet get inspired from those architectures that perform well in ILSVRCs. With the help of a large amount of face images with labels from public face recognition datasets [27], [43], [44], these CNN models are trained for learning most suitable face representations automatically for computer understanding and discrimination [57], and they get a high accuracy when testing on some specific datasets. The success of deep learning in the face recognition area motivates this project. However, the labelled data in the area of facial diagnosis is insufficient seriously. If we train a deep neural network from scratch, it will inevitably lead to overfitting. Apparently face recognition and facial diagnosis are related. Since the labelled data in the area of face recognition is much more, transfer learning technology comes into our view. In traditional learning, we train separate isolated models on specific datasets for different tasks. Transfer learning is to apply the knowledge gained while solving one problem to a different but related problem. According to whether the feature spaces of two domains are same or not, it can be divided into homogeneous transfer learning and heterogeneous transfer learning [38]. In our task, it belongs to homogeneous transfer learning. Deep transfer learning refers to transfer knowledge by deep neural networks. Thus, transfer learning makes it possible that identifying diseases from 2D face images by deep learning technique to provide a non-invasive and convenient way to realize early diagnosis and disease screening. In this paper, the next four diseases introduced and the corresponding health controls are selected to perform the validation.
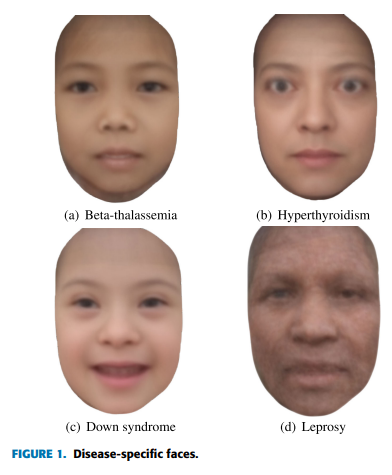
Thalassemia is a genetic disorder of blood caused by abnormal hemoglobin production, and it is one of the most common inherited blood disorders in the world. It is particularly common in people of Mediterranean, the Middle East, South Asian, Southeast Asian and Latin America. Since thalassemia can be fatal in early childhood without ongoing treatment, early diagnosis is vital for thalassemia. There are two different types of thalassemia: alpha (α) and beta (β). Beta-thalassemia is caused by mutations in the HBB gene which provides instructions for making a protein named beta-globin on chromosome 11, and is inherited in an autosomal recessive fashion. It is estimated that the annual incidence of symptomatic beta-thalassemia individuals worldwide is 1 in 100,000 [35]. According to medical research [13], beta-thalassemia can result in bone deformities, especially in the face. The typical characteristics of beta-thalassemia on the face include small eye openings, epicanthal folds, low nasal bridge, flat midface, short nose, smooth philtrum, thin upper lip and underdeveloped jaw (see Figure 1(a)). Hyperthyroidism is a common endocrine disease caused by excessive amounts of the thyroid hormones T3 and T4 which can regulate the body’s metabolism by various causes. The estimated average prevalence rate is 0.75% and the incidence rate is 51 per 100,000 persons per year by the meta-analysis [14]. If it is not treated early, hyperthyroidism will cause a series of serious complications and even threaten the patient’s life. The typical characteristics of hyperthyroidism on the face include thinning hair, shining and protruding or staring eyes, increased ocular fissure, less blinking, nervousness, consternation and fatigue.
The characteristic hyperthyroidism-specific face is shown as Figure 1(b). Down syndrome (DS) is a genetic disorder caused by the trisomy of chromosome 21. DS occurs in about one per one thousand the newborns each year. The common symptoms include physical growth delays, mild to moderate intellectual disability, and the special face. The typical characteristics of DS [15] on the face include larger head compared to the face, upward-slant of palpebral fissures, epicanthal folds, Brushfield spots, low-set small folded ears, flattened nasal bridge, short broad nose with depressed root and full tip, small oral cavity with broadened alveolar ridges and narrow palate, small chin and short neck. The characteristic DS-specific face is shown as Figure 1(c). Leprosy (also known Hansen’s disease) caused by a slow-growing type of bacteria named Mycobacterium leprae is an infectious disease. If the leper doesn’t accept timely treatment, leprosy will cause losing feelings of pain, weakness and poor eyesight. According to the World Health Organization, there are about 180,000 people infected with leprosy most of which are in Africa and Asia until 2017. The typical characteristics of leprosy [16] on the face include granulomas, hair loss, eye damage, pale areas of skin and facial disfigurement (e.g. loss of nose). The characteristic leprosy-specific face is shown as Figure 1(d). Identifying above diseases from uncontrolled 2D face images by deep learning technique has provided a good start for a non-invasive and convenient way to realize early diagnosis and disease screening. In this paper, our contributions are as follows: (1) We definitely propose using deep transfer learning from face recognition to perform the computer-aided facial diagnosis on various diseases. (2) We validate deep transfer learning methods for single and multiple diseases identification on a small dataset. (3) Through comparison, we find some rules for deep transfer learning from face recognition to facial diagnosis. The rest of this paper is organized as follows: Chapter 2 reviews the related work of computer-aided facial diagnosis. Chapter 3 describes our proposed methods and their implementations. Our experimental results are analyzed and discussed in Chapter 4. Chapter 5 makes a conclusion.
- RELATED WORK
Pan and Yang categorize transfer learning approaches into instance based transfer learning, feature based transfer learning, parameter based transfer learning, and relation based transfer learning [38]. Here we list some classical researches of each category. Instance based transfer learning is to reuse the source domain data by reweighting. Dai et al. presented TrAdaBoost to increase the instance weights that are beneficial to the target classification task and reduce the instance weights that are not conducive to the target classification task [45]. Tan et al. proposed a Selective Learning Algorithm (SLA) to solve the Distant Domain Transfer Learning (DDTL) problem with the supervised autoencoder as a base model for knowledge sharing among different domains [46]. As for feature based transfer learning, it is to encode the knowledge to be transferred into the learned feature representation to reduce the gap between the source domain and the target domain. Pan et al. presented transfer component analysis (TCA) using Maximum Mean Discrepancy (MMD) as the measurement criterion to minimize the data distribution difference in different domains [47]. Long et al. presented Joint Adaptation Networks (JAN) to align the joint distributions based on a joint maximum mean discrepancy (JMMD) criterion [48]. Regarding Parameter based transfer learning is to encode the transferred knowledge into the shared parameters. It is widely used in the medical application. Razavian et al. found that CNNs trained on large-scale datasets (e.g. ImageNet) are also pretty good feature extractors [49]. Esteva et al. used Google Inception v3 CNN architecture pretrained on the ImageNet dataset (1.28 million images over 1,000 generic object classes) and fine-tuned on their own dataset of 129,450 skin lesions comprising 2,032 different diseases [50]. The high accuracy demonstrates an artificial intelligence capable of classifying skin cancer with a level of competence comparable to dermatologists. Yu et al. used a voting system based on the output of three CNNs for medical images modality classification [51]. They fixed earlier layers of CNNs for reserving generic features of natural images, and trained high-level portion for medical image features. Shi et al. used a deep CNN based transfer learning method for pulmonary nodule detection in CT slices [52]. Raghu et al. demonstrated feature-independent benefits of transfer learning for better weight scaling and convergence speedups in medical imaging [53]. Shin et al. evaluated CNN architectures, dataset characteristics and transfer learning for thoraco-abdominal lymph node (LN) detection and interstitial lung disease (ILD) classification [54]. Besides, relation based transfer learning is to transfer the relationship among the data in the source and target domains. Davis and Domingos utilized Markov logic to discover properties of predicates including symmetry and transitivity, and relations among predicates [55]. In the following part, we review the previous researches on computer-aided facial diagnosis which are not many. Zhao et al. [15], [17], [18] used traditional machine learning methods for Down syndrome (DS) diagnosis with face images. Schneider et al. [19] performed detection of acromegaly by face classification which applied texture and geometry two principles to compare graphs for similarity. Kong et al. [20] performed detection of acromegaly from facial photographs by using the voting method to combine the predictions of basic estimators including Generalized Linear Models (GLM) [31], K-Nearest Neighbors (KNN), Support Vector Machines (SVM), CNN, and Random Forests (RF). Shu et al. [21] used eight extractors to extract texture features from face images and applied KNN and SVM classifiers to detect Diabetes Mellitus (DM).
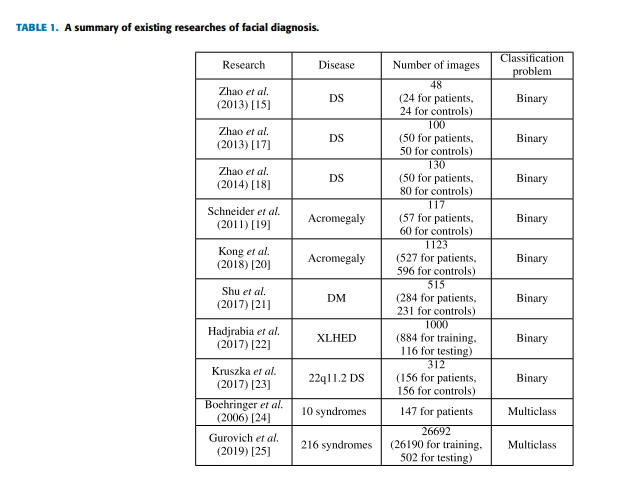
Hadj-Rabia et al. [22] detected the X-linked hypohidrotic ectodermal dysplasia (XLHED) phenotype from facial images with the Facial Dysmorphology Novel Analysis (FDNA) Software. Kruszka et al. [23] extracted 126 facial features including both geometric and texture biomarkers and used SVM classifiers to make 22q11.2 DS diagnoses. All the researches above [15], [17]–[23] performed binary classification with good results on the detection of one specific disease. But datasets of patients for testing are small comparing with ones of other applications. And most of them used handcraft features and traditional machine learning techniques. Boehringer et al. [24] achieved an over 75.7% classification accuracy for a computer-based diagnosis among the 10 syndromes by linear discriminant analysis (LDA) [32]. Gurovich et al. [25] developed a facial analysis framework named DeepGestalt which is trained with over 26,000 patient cases by fine-tuning a deep convolutional neural network (DCNN) to quantify similarities to different genetic syndromes. However, the multiclass classification tasks [24], [25] in facial diagnosis are with low top-1 accuracies, which are 75.7% and 60% correspondingly. Table 1 gives a brief summary of previous studies.
- MATERIALS AND METHODS
In this section, we describe the technology used in the method. For getting a better performance on the disease detection, sometimes we need a pre-processing procedure to remove interference factors to generate frontalized face images with a fixed size for the CNN input so that the performance of facial diagnosis can be improved. After getting the pre-processed inputs, we apply two strategies of deep transfer learning methods.
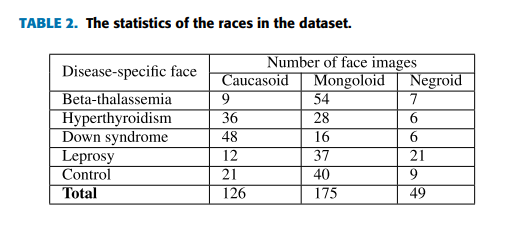
- DATASET
The Disease-Specific Face (DSF) dataset [9] used includes disease-specific face images which are collected from professional medical publications, medical forums, medical websites and hospitals with definite diagnostic results. In the task, there are totally 350 face images (JPG files) in the dataset, and there are 70 images in each type of disease-specific faces described in Chapter 1. Generally the ratio of training data and testing data is from 2:1 to 4:1. In our experiments with the small dataset, the ratio is set as 4:3 for the efficient evaluation. Table 2 shows the statistics of the races distinguished by eyes of face images in the experiments.
- PRE-PROCESSING
In the generally pre-processing procedure, we perform face detection on the original 2D face images by a face detector in OpenCV [26] which is based on Histogram of Oriented Gradients (HOG) features and a linear SVM classifier. The result of face detection is a bounding box containing the face located. Then, with the help of the Dlib library, we extract 68 facial landmarks [58] which are located on eyebrows, eyes, jaw lines, bridge and bottom of nose, edges of lips and chin to get the coordinate information. Next, with the help of 68 facial landmarks extracted we perform face alignment by using the affine transformation containing a series of transformations such as translation, rotation and scaling. Finally, the frontalized face image is cropped and resized according to the CNN used.
- DEEP TRANSFER LEARNING
Training a CNN which is end to end learning from scratch will inevitably lead to over-fitting since that the training data is generally insufficient for the task of facial diagnosis. Transfer learning is applying the knowledge gained while solving one problem to a different but related problem. In the transfer learning problem [33], generally we let Ds indicate the source domain, Dt indicate the target domain and X be the feature space domain. H is assumed to be a hypothesis class on X , and I(h) is the set for characteristic function h ∈ H. The definition of H-divergence between Ds and Dt which is used to estimate divergence of unlabeled data is:

where Pr indicates the probability distribution. Furthermore, the relationship between errors of target domain and source domain can be calculated as:
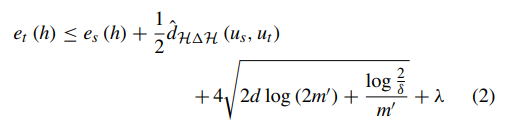
where us and ut are unlabeled samples from Ds and Dt respectively. For briefly, the difference in error between source domain and task domain is bounded as:

where dH1H indicates the distance of symmetric difference hypothesis space H1H. The equations above have proved that transfer learning from different domains is mathematically effective [34]. Deep transfer learning (DTL) [38], [39] is to transfer knowledge by pretrained deep neural network which originally aims to perform facial verification and recognition in this paper. Thus the source task is face recognition and verification, and the target task is facial diagnosis. In this case, the feature spaces of the source domain and target domain are same while the source task and the target task are different but related. The similarity of two tasks motivates us to use deep transfer learning from face recognition to solve facial diagnosis problem with a small dataset. If divided according to transfer learning scenarios, it belongs to inductive transfer learning. If divided according to transfer learning methods, it belongs to parameter based transfer learning. In this section, two main deep transfer learning strategies [40], [41] are applied to perform comparison. In the main experiment, DCNN models pretrained by VGG-Face dataset [27] and ImageNet dataset [42] are compared with traditional machine learning methods. VGG-Face dataset contains 2.6M images over 2.6K people for face recognition and verification, and ImageNet dataset contains more than 14M images of 20K categories for visual object recognition. The pretrained CNN is for end-to-end learning so that it can extract high-level features automatically. Since deep transfer learning is based on the fact that CNN features are more generic in early layers and more original dataset-specific in later layers, operation should be performed on the last layers of DCNN models. The diagram of facial diagnosis by deep transfer learning is shown in Figure 2. The implementation is based on Matlab (version: 2017b) with its CNNs toolbox for computer vision applications named MatConvNet (version: 1.0-beta25). NVIDIA CUDA toolkit (version: 9.0.176) and its library CuDNN (version: 7.4.1) are applied for GPU (model: Nvidia GeForce GTX 1060) accelerating.
- DTL1: FINE-TUNING THE PRETRAINED CNN MODEL
In this section, we replace the final fully connected layer of the pretrained CNN by initializing the weight. When fine-tuning the CNN (see Pseudocode 1), we calculate activation value through forward propagation of the convolutional layer as:

where a indicates input feature map of some layer, and k indicates its corresponding kernel. σ is defined as:

Therefore, the output value of convolution operation is calculated as f (c l u,v ) in which f is the activation function. When updating the weights, we calculate error term through back propagation of the convolutional layer as:
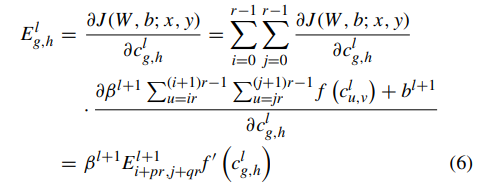
where f , same with above, represents the activation function, J represents the cost function, (W, b) are the parameters and
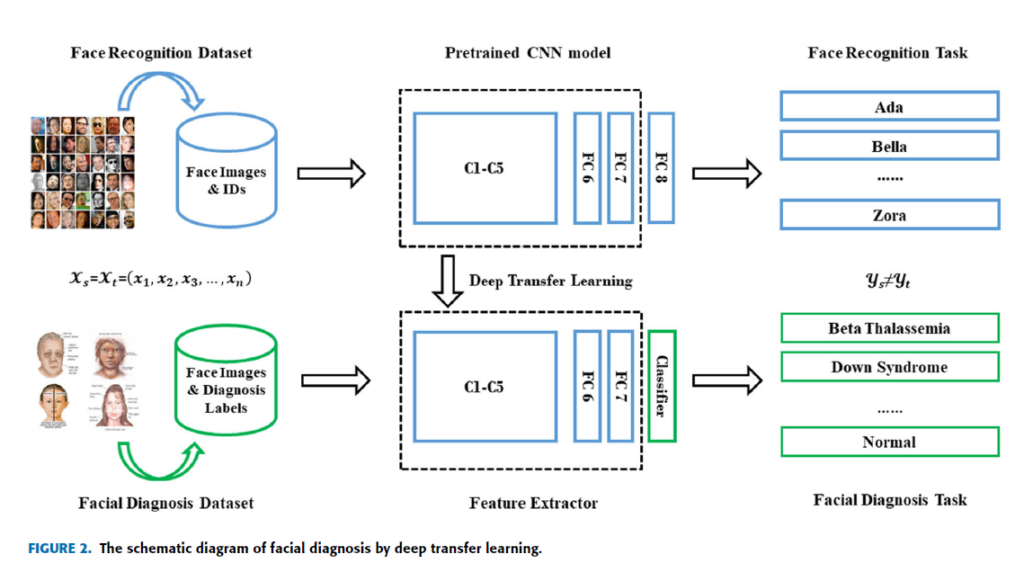
(x, y) are the training data and label pairs. Since the pretrained model has already converged on the original training data, a small learning rate of 5 × 10−5 is utilized. Weight Decay for avoiding overfitting to a certain extent is set as 5 × 10−4 , and momentum for accelerating convergence in mini-batch gradient descent (SGD) is set as 0.9. Here we take VGG-16 model also named VGG-Face as an example, which is the best case in the main experiment. A softmax loss layer is added for retraining by 100 epochs initially. Figure 3 containing three indicators Objective, Top-1 error and Top-3 error shows the process of fine-tuning the pretrained VGG-Face for the multiclass classification task. Objective is the sum loss of all samples in a batch. The loss can be calculated as:

where yi refers to the i th true classification result, pi represents the i th output of the softmax function, and zi represents the i th output of the convolutional neural network. The Top-1 error refers to the percentage of the time that the classifier did not correctly predict the class with the highest score. The Top-3 error refers to the percentage of the time that the classifier did not include the correct class among its top 3 guesses. As it can be seen from Figure 3, all three indicators converge after retraining about 11 epochs, which indicates fine-tuning is successful and effective. However, the validation error is higher than the training error, which is because of the limitation of the fine-tuning strategy on the small dataset. As shown in Figure 3, after 24 epochs the validation top-1 error rises while the training error doesn’t, which indicates over-fitting may occur. So we saved the fine-tuned CNN model after retraining 24 epochs for testing. The early stopping technique is used here. The softmax layer is used for classification, which is consistent with the pretrained model. Time complexity is the number of calculations of one model/algorithm, which can be measured with floating point operations (FLOPs). In our estimations, the Multiply-Accumulate Operation (MAC) is used as the unit of FLOPs. In CNNs, time complexity of a single convolutional layer can be estimated as:

where M is the side length of the feature map output by each kernel, K is the side length of each kernel, and C represents the number of corresponding channels [59]. Thus, the overall time complexity of convolutional neural networks can be estimated as:
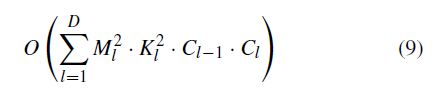
The FLOPs of the fully connected layers can be estimated by I · O where I indicates input neuron numbers and O indicates output neuron numbers. I corresponds to Cl−1 and O corresponds to Cl in the above formula. Because pretrained models for object and face recognition have a larger number of categories, the time complexity of adapted models by DTL1 in our task is smaller than the original corresponding pretrained model.
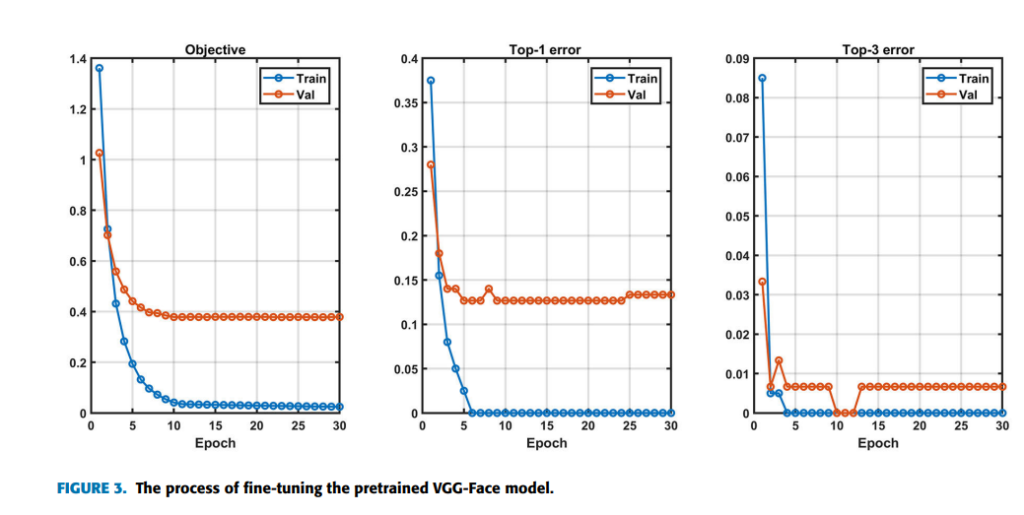
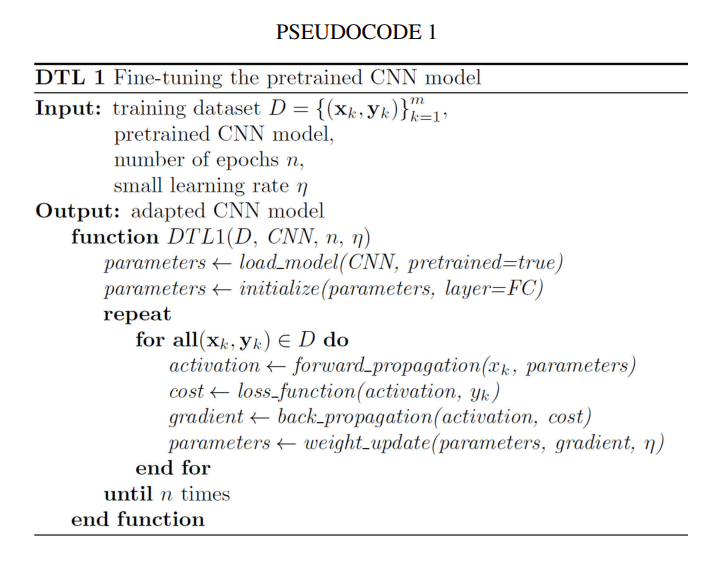
- DTL2: CNN AS FIXED FEATURE EXTRACTOR
In this section, the CNN is used as a feature extractor directly for the smaller dataset (see Pseudocode 2). During training process for facial diagnosis, we only want to utilize the partial weighted layers of the pretrained CNN model to extract features, but not to update the weights of it. As the architect Ludwig Mies van der Rohe said, ‘‘Less is more’’. We select the linear kernel for the SVM [37] model to do classification in this strategy, because the dimension of the input feature vectors is much larger than the number of samples. For the reason that CNN features are more original dataset specific in the last layers, we directly extract features of the layer which is located before the final fully connected layer of pretrained DCNN models, and then train a linear SVM classifier leveraging the features extracted as:

where C which is a hyper-parameter indicates a penalty factor, and (xi, yi) represents the training data. After the training process, we could obtain the linear SVM model trained to perform testing. During the training phase, the time complexity of SVM is different in different situations, namely whether most support vectors are at the upper bound or not, and depending on the ratio of the number of vectors and the number of training points. During the testing phase, the time complexity of SVM is O(M · Ns) where M is the number of operations required by the corresponding kernel, and Ns is the number of support vectors. For a linear SVM classifier, the algorithm complexity is O(dl · Ns) where dl is the dimension of input vectors [56]. In our tasks, Ns is larger than the number of output neurons of CNN final fully connected layers in DTL1, while generally smaller than it in the original corresponding pretrained models.
- RESULTS AND DISCUSSIONS
In this section, we perform the experiments on two tasks of facial diagnosis by two strategies of deep transfer learning including fine-tuning abbreviated as DTL1 and using CNN as a feature extractor abbreviated as DTL2. The deep learning models pretrained for object detection and face recognition are selected for comparison. In addition, we compare the results with traditional machine learning methods using the hand-crafted feature that is Dense Scale Invariant Feature Transform (DSIFT) [28]. DSIFT, which is often used in object recognition, performs Scale Invariant
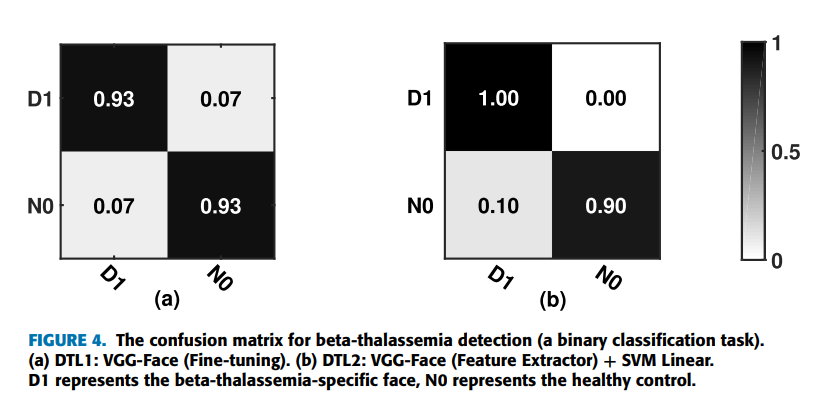
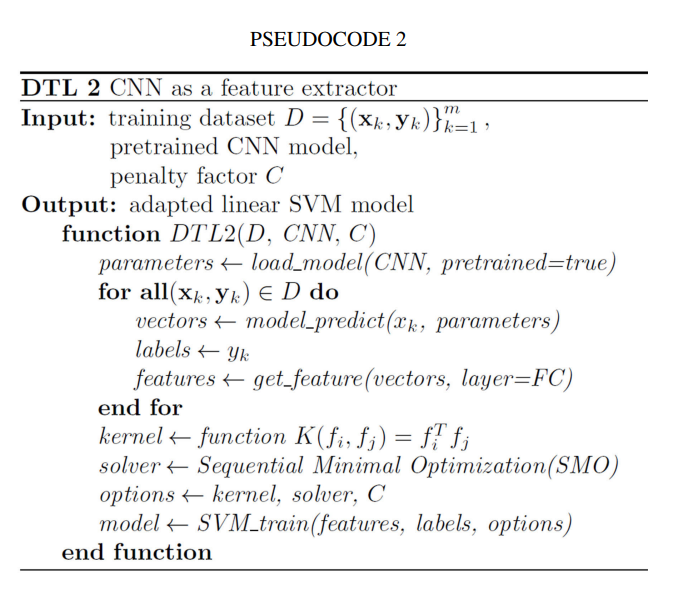
Feature Transform (SIFT) on a dense gird of locations of the image at a certain scale and orientation. The SVM algorithm for its good performance in few-shot learning is used as the classifier for Bag of Features (BOF) models with DSIFT descriptors. Two cases of facial diagnosis are designed in this paper. One is the detection of beta-thalassemia, which is a binary classification task. The other one is the detection of four diseases which are beta-thalassemia, hyperthyroidism, Down syndrome and leprosy with the healthy control, which is a multiclass classification task and more challenging.
- SINGLE DISEASE DETECTION (BETA-THALASSEMIA): A BINARY CLASSIFICATION TASK
In practical, we usually need to perform detection or screening on one specific disease. In this case, we only use 140 images of the dataset which are 70 betathalassemia-specific face images and 70 images for healthy control. 40 of each type images are for training, and 30 of each type images are for testing. It is a binary classification task. By comparing all selected machine learning methods (see Table 3), we find that the best overall top-1 accuracies
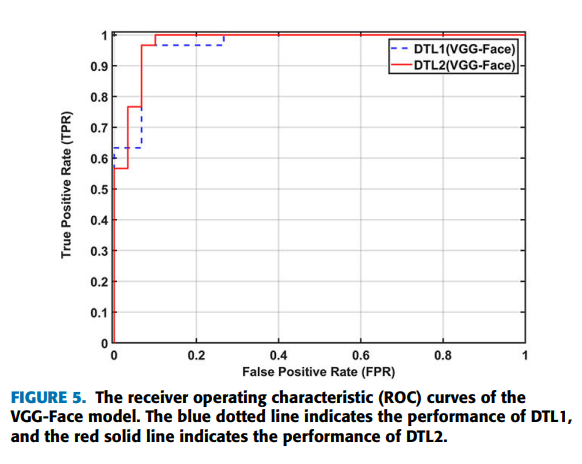
can be achieved by using the strategies of deep transfer learning on the VGG-Face model (VGG-16 pretrained on the VGG-Face dataset). Furthermore, applying DTL2: CNN as a feature extractor can get a better accuracy of 95.0% than using DTL1: fine-tuning in this task, which is indicated by Figure 4. Figure 4 shows the confusion matrices of DTL1 and DTL2 on the VGG-Face model in this task. D1 represents the beta-thalassemia-specific face, and N0 represents the healthy control. The row in the confusion matrix indicates the predicted classes, and the column in the confusion matrix indicates the actual classes. In detail, two of thirty testing images for each type, false positives and false negatives, are misclassified by DTL1, which leads to an accuracy of 93.3%. For DTL2, thirty images belonging to the type of beta-thalassemia in actual, true positives, are all classified correctly. On the other hand, three of thirty images, false positives, are belonging to the healthy control in actual, but classified as the beta-thalassemia-specific face. Figure 5 shows the receiver operating characteristic (ROC) curves of the VGG-Face model by DTL1 and DTL2. The blue dotted line indicates the performance of DTL1, and the red solid line indicates the performance of DTL2. The Areas Under ROC curves (AUC) calculated are 0.969 and 0.978 correspondingly.
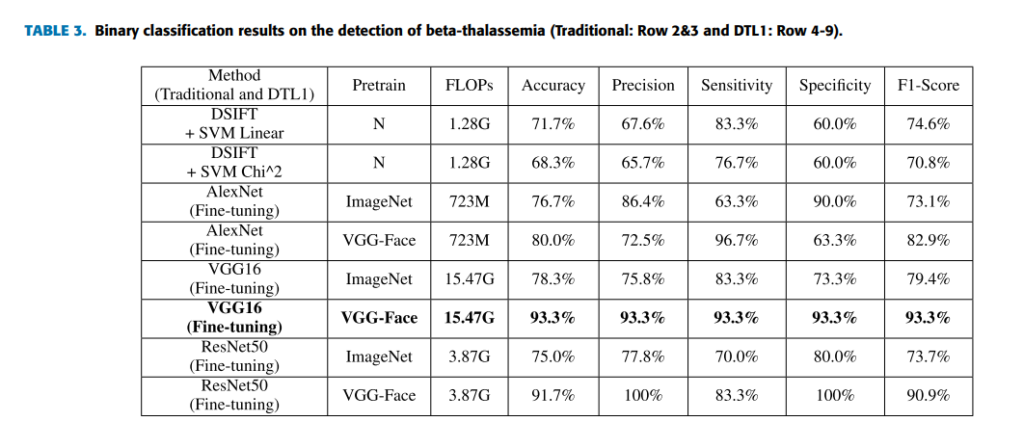
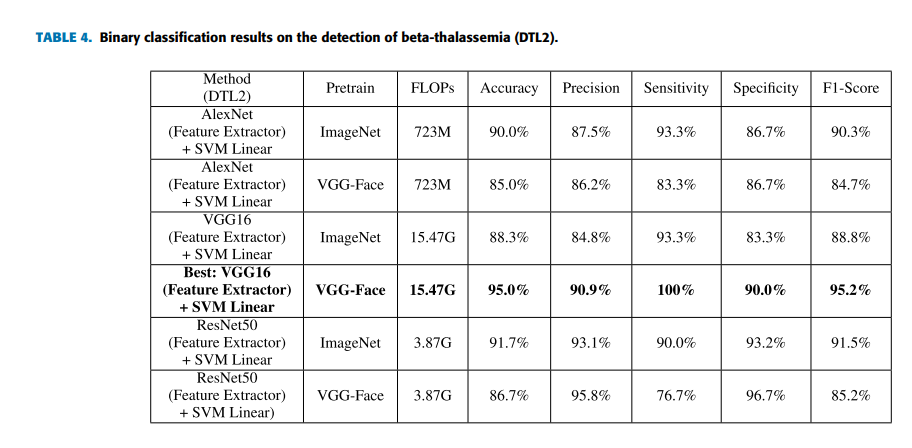
For comparison, deep learning models pretrained such as AlexNet, VGG16 and ResNet are used. In addition, traditional machine learning methods extracting DSIFT features on the face image and predicting with a linear or nonlinear SVM classifier [29] are selected. Five indicators that are accuracy, precision, sensitivity, specificity and F1-score which is a weighted average of the precision and sensitivity are selected to evaluate the performance of models. The indicator of FLOPs spent for forward pass is estimated to evaluate the time complexity of models. Table 3 lists the results of both traditional machine learning methods and fine-tuning deep learning models pretrained on the ImageNet and VGG-Face dataset in this task. From the results, we find that the performance by traditional machine learning methods is close to the performance of fine-tuning (DTL1) deep learning models pretrained on ImageNet. However, the performance of fine-tuning (DTL1) the deep learning models pretrained on VGG-Face is overall better than ones pretrained on ImageNet, which is reasonable. Because the source domain of VGG-Face is nearer to DSF dataset than ImageNet. Table 4 lists the results of CNN as a feature extractor on the pretrained deep learning models (DTL2). Applying DTL2: CNN as a feature extractor can get an overall better performance than traditional machine learning methods and DTL1. However, deep learning models pretrained on VGG-Face seem to behave not necessarily better than deep learning models pretrained on ImageNet in this strategy. It will be investigated further in the next experiment.
- VARIOUS DISEASES DETECTION: A MULTICLASS CLASSIFICATION TASK
In practical, that we perform various diseases detection or screening at one time could greatly increase efficiency. For evaluating the algorithm further, in this case there are totally 350 images in the task dataset, and there are 70 images for each type of faces. For the training process, totally 200 images (40 images of each type) are used. For the testing process, totally 150 images (30 images of each type) are used. It is a multiclass classification task. By comparing
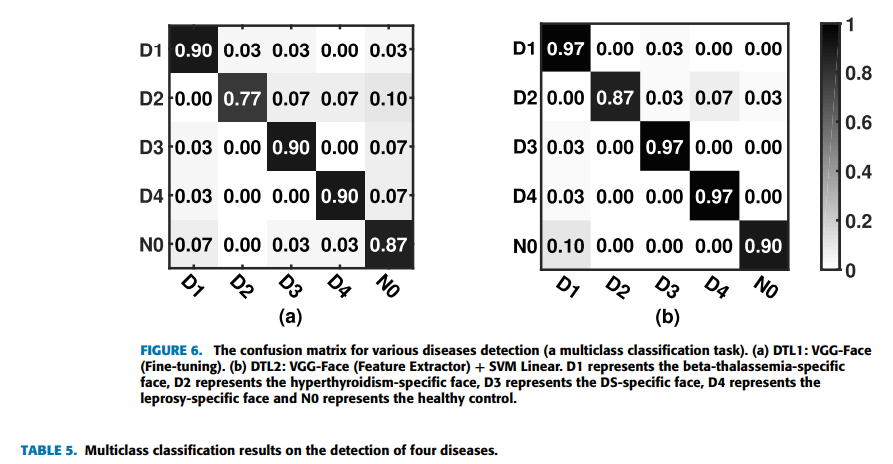
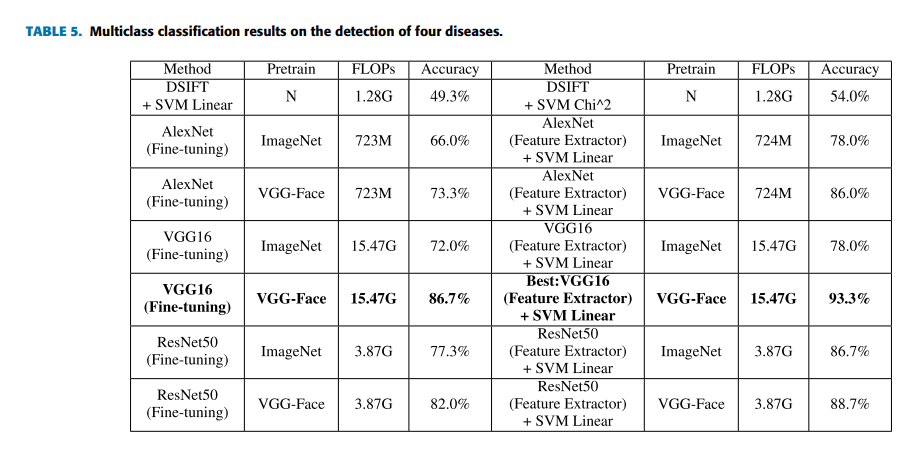
all selected machine learning methods, we find that the best overall top-1 accuracies can be achieved by using the strategies of deep transfer learning on the VGG-Face model again. Furthermore, applying DTL2: VGG-Face as a feature extractor can get a better accuracy of 93.3% than using DTL1: fine-tuning in this task, which is indicated by Figure 6. Figure 6 shows the confusion matrices of DTL1 and DTL2 on the VGG-Face model in this task. D1 represents the beta-thalassemia-specific face, D2 represents the hyperthyroidism-specific face, D3 represents the DS-specific face, D4 represents the leprosy-specific face and N0 represents the healthy control. The row in the confusion matrix indicates the predicted classes, and the column in the confusion matrix indicates the actual classes. From the Figure 6(b), four of thirty images are belonging to the hyperthyroidism-specific face in actual, but classified as other types, which indicates it is relatively difficult for the classifier to recognize hyperthyroidism from face images. For recognizing beta-thalassemia, Down syndrome and leprosy, the classifier has a very good accuracy. Figure 6(a) of DTL1 also shows a low accuracy on recognizing hyperthyroidism. Table 5 lists the results of traditional machine learning methods and deep learning methods in the multiclass classification task as described before. Since the multiclass classification task is more difficult than the binary classification task before, the accuracies of machine learning models decrease generally. The results by deep transfer learning methods are much better than the results by traditional machine learning methods in this task, which is as expected. And deep learning models pretrained on VGG-Face behave generally better than deep learning models pretrained on ImageNet in both strategies. The performance of DTL2: CNN as a feature extractor is overall better than that of DTL1:
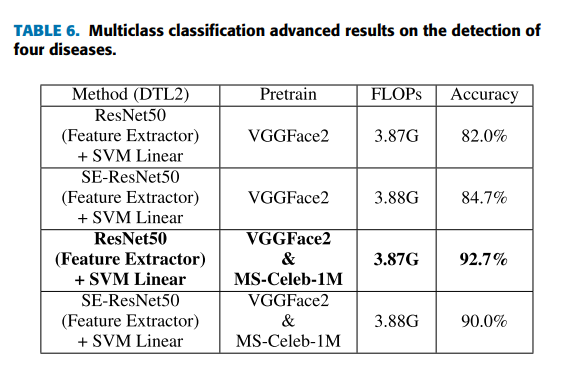
Fine-tuning again, which probably is due to the relatively small dataset. On the basis of applying DTL2, for exploring a better performance by deep transfer learning, we investigate the performance of ResNet50 and SE-ResNet50 [36] models pretrained on MS-Celeb-1M [43] and VGGFace2 [44]. MS-Celeb-1M is a widely used dataset of roughly 10 million photos from 100,000 individuals for face recognition. VGGFace2 is a large-scale dataset containing more than 3.3 million face images over 9K identities for face recognition. Table 6 lists the results of ResNet50 and SE-ResNet50 models pretrained on the different datasets. SE-ResNet50 has more complex structure but does not get better results than ResNet50 here, which accords with the fact that ‘‘VGG-Face’’ model achieves the best results in our experiments. The results indicate pretraining on more task-related datasets can improve the performance in this task. The ResNet50 pretrained on MS-Celeb-1M and finetuned on VGGFace2 improves its accuracy from 86.7% (ImageNet) to 92.7% which is closest to the best result. In addition, clinicians from Jiangsu Province Hospital and Zhongda Hospital Affiliated To Southeast University are invited to perform the detection on the same task to get an average accuracy of 84.5%, which is similar with the accuracy of the specialists published before [23]. DTL2: CNN as a feature extractor still outperforms clinicians, which is promising. Regarding the time complexity (see Table 3-6), as mentioned in the theoretical part, the time complexity of DTL1 and DTL2 are both smaller than that of the corresponding pretrained model, and the time complexity of DTL2 is a bit larger than that of DTL1. Since the FLOPs of CNN models are almost more than a few hundred millions now, the difference in FLOPs values of the adapted model and its corresponding pretrained model shown in tables is not obvious. From these experiments, we can conclude that the performance by deep learning methods are overall better than the results by traditional machine learning methods as expected. The difference is more expressive for the multiclass classification task. In the case of the small dataset of facial diagnosis, DTL2: CNN as a feature extractor is more appropriate than DTL1: Fine-tuning. Furthermore, it is because of the similarity between the target domain and the source domain of deep learning models pretrained for face recognition that the better performance can be reached by deep transfer learning methods. Deep learning models pretrained on more datasets for face recognition can achieve a better performance on facial diagnosis by deep transfer learning.
- CONCLUSION
More and more studies have shown that computer-aided facial diagnosis is a promising way for disease screening and detection. In this paper, we propose deep transfer learning from face recognition methods to realize computer-aided facial diagnosis definitely and validate them on single disease and various diseases with the healthy control. The experimental results of above 90% accuracy have proven that CNN as a feature extractor is the most appropriate deep transfer learning method in the case of the small dataset of facial diagnosis. It can solve the general problem of insufficient data in the facial diagnosis area to a certain extent. In future, we will continue to discover deep learning models to perform facial diagnosis effectively with the help of data augmentation methods. We hope that more and more diseases can be detected efficiently by face photographs.
ACKNOWLEDGMENT
The Visual Information Security (VIS) Team supports us theoretically and technically. The authors would like to thank all the members of VIS team. They also would like to thank Professors Urbano José Carreira Nunes, Helder Jesus Araújo and Rui Alexandre Matos Araújo for their valuable suggestions.
REFERENCES
[1] P. U. Unschuld, Huang Di Nei Jing Su Wen: Nature, Knowledge, Imagery in an Ancient Chinese Medical Text: With an Appendix: The Doctrine of the Five Periods and Six Qi in the Huang Di Nei Jing Su Wen. Univ of California Press, 2003.
[2] A. Krizhevsky, I. Sutskever, and G. E. Hinton, ‘‘Imagenet classification with deep convolutional neural networks,’’ in Proc. Adv. Neural Inf. Process. Syst., 2012, pp. 1097–1105.
[3] C. Szegedy, W. Liu, Y. Jia, P. Sermanet, S. Reed, D. Anguelov, D. Erhan, V. Vanhoucke, and A. Rabinovich, ‘‘Going deeper with convolutions,’’ in Proc. IEEE Conf. Comput. Vis. Pattern Recognit. (CVPR), Jun. 2015, pp. 1–9.
[4] K. Simonyan and A. Zisserman, ‘‘Very deep convolutional networks for large-scale image recognition,’’ 2014, arXiv:1409.1556. [Online]. Available: http://arxiv.org/abs/1409.1556
[5] K. He, X. Zhang, S. Ren, and J. Sun, ‘‘Deep residual learning for image recognition,’’ in Proc. IEEE Conf. Comput. Vis. Pattern Recognit. (CVPR), Jun. 2016, pp. 770–778.
[6] C. Szegedy, S. Ioffe, V. Vanhoucke, and A. A. Alemi, ‘‘Inception-v4, inception-resnet and the impact of residual connections on learning,’’ in Proc. 31st AAAI Conf. Artif. Intell., 2017, pp. 1–12.
[7] F. Schroff, D. Kalenichenko, and J. Philbin, ‘‘FaceNet: A unified embedding for face recognition and clustering,’’ in Proc. IEEE Conf. Comput. Vis. Pattern Recognit. (CVPR), Jun. 2015, pp. 815–823.
[8] Y. Taigman, M. Yang, M. Ranzato, and L. Wolf, ‘‘DeepFace: Closing the gap to human-level performance in face verification,’’ in Proc. IEEE Conf. Comput. Vis. Pattern Recognit., Jun. 2014, pp. 1701–1708.
[9] B. Jin, ‘‘Disease-specific faces,’’ IEEE Dataport, 2020. Accessed: Jun. 29, 2020. [Online]. Available: http://dx.doi.org/10.21227/ rk2v-ka85
[10] J. Liu, Y. Deng, T. Bai, Z. Wei, and C. Huang, ‘‘Targeting ultimate accuracy: Face recognition via deep embedding,’’ 2015, arXiv:1506.07310. [Online]. Available: http://arxiv.org/abs/1506.07310
[11] J. Fanghänel, T. Gedrange, and P. Proff, ‘‘The face-physiognomic expressiveness and human identity,’’ Ann. Anatomy-Anatomischer Anzeiger, vol. 188, no. 3, pp. 261–266, May 2006.
[12] B. Zhang, X. Wang, F. Karray, Z. Yang, and D. Zhang, ‘‘Computerized facial diagnosis using both color and texture features,’’ Inf. Sci., vol. 221, pp. 49–59, Feb. 2013.
[13] E. S. J. A. Alhaija and F. N. Hattab, ‘‘Cephalometric measurements and facial deformities in subjects with -thalassaemia major,’’ Eur. J. Orthodontics, vol. 24, no. 1, pp. 9–19, Feb. 2002.
[14] P. N. Taylor, D. Albrecht, A. Scholz, G. Gutierrez-Buey, J. H. Lazarus, C. M. Dayan, and O. E. Okosieme, ‘‘Global epidemiology of hyperthyroidism and hypothyroidism,’’ Nature Rev. Endocrinol., vol. 14, no. 5, pp. 301–316, 2018.
[15] Q. Zhao, K. Rosenbaum, R. Sze, D. Zand, M. Summar, and M. G. Linguraru, ‘‘Down syndrome detection from facial photographs using machine learning techniques,’’ Proc. SPIE, vol. 8670, Feb. 2013, Art. no. 867003.
[16] E. Turkof, B. Khatri, S. Lucas, O. Assadian, B. Richard, and E. Knolle, ‘‘Leprosy affects facial nerves in a scattered distribution from the main trunk to all peripheral branches and neurolysis improves muscle function of the face,’’ Amer. J. Tropical Med. Hygiene, vol. 68, no. 1, pp. 81–88, Jan. 2003.
[17] Q. Zhao, K. Okada, K. Rosenbaum, D. J. Zand, R. Sze, M. Summar, and M. G. Linguraru, ‘‘Hierarchical constrained local model using ICA and its application to Down syndrome detection,’’ in Proc. Int. Conf. Med. Image Comput. Comput.-Assist. Intervent. Berlin, Germany: Springer, 2013, pp. 222–229.
[18] Q. Zhao, K. Okada, K. Rosenbaum, L. Kehoe, D. J. Zand, R. Sze, M. Summar, and M. G. Linguraru, ‘‘Digital facial dysmorphology for genetic screening: Hierarchical constrained local model using ICA,’’ Med. Image Anal., vol. 18, no. 5, pp. 699–710, Jul. 2014.
[19] H. J. Schneider, R. P. Kosilek, M. Günther, J. Roemmler, G. K. Stalla, C. Sievers, M. Reincke, J. Schopohl, and R. P. Würtz, ‘‘A novel approach to the detection of acromegaly: Accuracy of diagnosis by automatic face classification,’’ J. Clin. Endocrinol. Metabolism, vol. 96, no. 7, pp. 2074–2080, Jul. 2011.
[20] X. Kong, S. Gong, L. Su, N. Howard, and Y. Kong, ‘‘Automatic detection of acromegaly from facial photographs using machine learning methods,’’ EBioMedicine, vol. 27, pp. 94–102, Jan. 2018.
[21] T. Shu, B. Zhang, and Y. Y. Tang, ‘‘An extensive analysis of various texture feature extractors to detect diabetes mellitus using facial specific regions,’’ Comput. Biol. Med., vol. 83, pp. 69–83, Apr. 2017.
[22] S. Hadj-Rabia, H. Schneider, E. Navarro, O. Klein, N. Kirby, K. Huttner, L. Wolf, M. Orin, S. Wohlfart, C. Bodemer, and D. K. Grange, ‘‘Automatic recognition of the XLHED phenotype from facial images,’’ Amer. J. Med. Genet. Part A, vol. 173, no. 9, pp. 2408–2414, Sep. 2017.
[23] P. Kruszka, Y. A. Addissie, D. E. McGinn, A. R. Porras, E. Biggs, M. Share, and T. B. Crowley, ‘‘22q11. 2 deletion syndrome in diverse populations,’’ Amer. J. Med. Genetics A, vol. 173, no. 4, pp. 879–888, 2017. [24] S. Boehringer, T. Vollmar, C. Tasse, R. P. Wurtz, G. Gillessen-Kaesbach, B. Horsthemke, and D. Wieczorek, ‘‘Syndrome identification based on 2D analysis software,’’ Eur. J. Hum. Genet., vol. 14, no. 10, pp. 1082–1089, Oct. 2006.
[25] Y. Gurovich, Y. Hanani, O. Bar, G. Nadav, N. Fleischer, D. Gelbman, L. Basel-Salmon, P. M. Krawitz, S. B. Kamphausen, M. Zenker, L. M. Bird, and K. W. Gripp, ‘‘Identifying facial phenotypes of genetic disorders using deep learning,’’ Nature Med., vol. 25, no. 1, pp. 60–64, Jan. 2019.
[26] G. Bradski and A. Kaehler, Learning OpenCV: Computer Vision With the OpenCV Library. Newton, MA, USA: O’Reilly Media, 2008.
[27] O. M. Parkhi, A. Vedaldi, and A. Zisserman, ‘‘Deep face recognition,’’ in Proc. Brit. Mach. Vis. Conf., 2015, pp. 1–12. [28] J.-G. Wang, J. Li, C. Y. Lee, and W.-Y. Yau, ‘‘Dense SIFT and Gabor descriptors-based face representation with applications to gender recognition,’’ in Proc. 11th Int. Conf. Control Autom. Robot. Vis., Dec. 2010, pp. 1860–1864.
[29] C. Shan, S. Gong, and P. W. McOwan, ‘‘Robust facial expression recognition using local binary patterns,’’ in Proc. IEEE Int. Conf. Image Process., Sep. 2005. pp. II–370.
[30] D. Wu, Y. Chen, C. Xu, K. Wang, H. Wang, F. Zheng, D. Ma, and G. Wang, ‘‘Characteristic face: A key indicator for direct diagnosis of 22q11. 2 deletions in Chinese velocardiofacial syndrome patients,’’ PLoS ONE, vol. 8, no. 1, 2013, Art. no. e54404.
[31] J. Wen, Y. Xu, Z. Li, Z. Ma, and Y. Xu, ‘‘Inter-class sparsity based discriminative least square regression,’’ Neural Netw., vol. 102, pp. 36–47, Jun. 2018.
[32] J. Wen, X. Fang, J. Cui, L. Fei, K. Yan, Y. Chen, and Y. Xu, ‘‘Robust sparse linear discriminant analysis,’’ IEEE Trans. Circuits Syst. Video Technol., vol. 29, no. 2, pp. 390–403, Feb. 2019, doi: 10.1109/TCSVT.2018.2799214.
[33] S. Ben-David, J. Blitzer, K. Crammer, A. Kulesza, F. Pereira, and J. W. Vaughan, ‘‘A theory of learning from different domains,’’ Mach. Learn., vol. 79, nos. 1–2, pp. 151–175, May 2010.
[34] S. Ben-David, J. Blitzer, K. Crammer, and F. Pereira, ‘‘Analysis of representations for domain adaptation,’’ in Proc. Adv. Neural Inf. Process. Syst., 2007, pp. 137–144.
[35] R. Galanello and R. Origa, ‘‘Beta-thalassemia,’’ Orphanet J. Rare Diseases, vol. 5, no. 1, p. 11, 2010. [36] J. Hu, L. Shen, and G. Sun, ‘‘Squeeze-and-Excitation networks,’’ in Proc. IEEE/CVF Conf. Comput. Vis. Pattern Recognit., Jun. 2018, pp. 7132–7141.
[37] J. A. K. Suykens and J. Vandewalle, ‘‘Least squares support vector machine classifiers,’’ Neural Process. Lett., vol. 9, no. 3, pp. 293–300, Jun. 1999.
[38] S. J. Pan and Q. Yang, ‘‘A survey on transfer learning,’’ IEEE Trans. Knowl. Data Eng., vol. 22, no. 10, pp. 1345–1359, Oct. 2010.
[39] L. Shao, F. Zhu, and X. Li, ‘‘Transfer learning for visual categorization: A survey,’’ IEEE Trans. Neural Netw. Learn. Syst., vol. 26, no. 5, pp. 1019–1034, May 2015.
[40] D. Sarkar, A Comprehensive Hands-on Guide to Transfer Learning With Real-World Applications in Deep Learning. Medium, 2018.
[41] S. Ruder. Transfer Learning-Machine Learning’s Next Frontier. Accessed: 2017. [Online]. Available: https://ruder.io/transfer-learning/
[42] J. Deng, W. Dong, R. Socher, L.-J. Li, K. Li, and L. Fei-Fei, ‘‘ImageNet: A large-scale hierarchical image database,’’ in Proc. IEEE Conf. Comput. Vis. Pattern Recognit., Jun. 2009, pp. 248–255.
[43] Y. Guo, L. Zhang, Y. Hu, X. He, and J. Gao, ‘‘MS-Celeb-1M: A dataset and benchmark for large-scale face recognition,’’ in Proc. Eur. Conf. Comput. Vis. Cham, Switzerland: Springer, 2016, pp. 87–102.
[44] Q. Cao, L. Shen, W. Xie, O. M. Parkhi, and A. Zisserman, ‘‘VGGFace2: A dataset for recognising faces across pose and age,’’ in Proc. 13th IEEE Int. Conf. Autom. Face Gesture Recognit. (FG), May 2018, pp. 67–74.
[45] W. Dai, Q. Yang, G.-R. Xue, and Y. Yu, ‘‘Boosting for transfer learning,’’ in Proc. 24th Int. Conf. Mach. Learn. (ICML), 2007, pp. 193–200.
[46] B. Tan, Y. Zhang, S. J. Pan, and Q. Yang, ‘‘Distant domain transfer learning,’’ in Proc. 31st AAAI Conf. Artif. Intell., 2017, pp. 2604–2610.
[47] S. J. Pan, I. W. Tsang, J. T. Kwok, and Q. Yang, ‘‘Domain adaptation via transfer component analysis,’’ IEEE Trans. Neural Netw., vol. 22, no. 2, pp. 199–210, Feb. 2011.
[48] M. Long, H. Zhu, J. Wang, and M. I. Jordan, ‘‘Deep transfer learning with joint adaptation networks,’’ in Proc. 34th Int. Conf. Mach. Learn., Vol. 70, 2017, pp. 2208–2217.
[49] A. S. Razavian, H. Azizpour, J. Sullivan, and S. Carlsson, ‘‘CNN features Off-the-shelf: An astounding baseline for recognition,’’ in Proc. IEEE Conf. Comput. Vis. Pattern Recognit. Workshops, Jun. 2014, pp. 806–813.
[50] A. Esteva, B. Kuprel, R. A. Novoa, J. Ko, S. M. Swetter, H. M. Blau, and S. Thrun, ‘‘Dermatologist-level classification of skin cancer with deep neural networks,’’ Nature, vol. 542, no. 7639, pp. 115–118, Feb. 2017.
[51] Y. Yu, H. Lin, J. Meng, X. Wei, H. Guo, and Z. Zhao, ‘‘Deep transfer learning for modality classification of medical images,’’ Information, vol. 8, no. 3, p. 91, Jul. 2017.
[52] Z. Shi, H. Hao, M. Zhao, Y. Feng, L. He, Y. Wang, and K. Suzuki, ‘‘A deep CNN based transfer learning method for false positive reduction,’’ Multimedia Tools Appl., vol. 78, no. 1, pp. 1017–1033, Jan. 2019. [53] M. Raghu, C. Zhang, J. Kleinberg, and S. Bengio, ‘‘Transfusion: Understanding transfer learning for medical imaging,’’ in Proc. Adv. Neural Inf. Process. Syst., 2019, pp. 3342–3352.
[54] H.-C. Shin, H. R. Roth, M. Gao, L. Lu, Z. Xu, I. Nogues, J. Yao, D. Mollura, and R. M. Summers, ‘‘Deep convolutional neural networks for computer-aided detection: CNN architectures, dataset characteristics and transfer learning,’’ IEEE Trans. Med. Imag., vol. 35, no. 5, pp. 1285–1298, May 2016.
[55] J. Davis and P. Domingos, ‘‘Deep transfer via second-order Markov logic,’’ in Proc. 26th Annu. Int. Conf. Mach. Learn. (ICML), 2009, pp. 217–224.
[56] C. J. C. Burges, ‘‘A tutorial on support vector machines for pattern recognition,’’ Data Mining Knowl. Discovery, vol. 2, no. 2, pp. 121–167, 1998.
[57] A. F. Abate, P. Barra, S. Barra, C. Molinari, M. Nappi, and F. Narducci, ‘‘Clustering facial attributes: Narrowing the path from soft to hard biometrics,’’ IEEE Access, vol. 8, pp. 9037–9045, 2020.
[58] V. Kazemi and J. Sullivan, ‘‘One millisecond face alignment with an ensemble of regression trees,’’ in Proc. IEEE Conf. Comput. Vis. Pattern Recognit., Jun. 2014, pp. 1867–1874. [59] P. Molchanov, S. Tyree, T. Karras, T. Aila, and J. Kautz, ‘‘Pruning convolutional neural networks for resource efficient inference,’’ 2016, arXiv:1611.06440. [Online]. Available: http://arxiv.org/abs/1611.06440