© 2018Korea Institute of Oriental Medicine.Publishing services by Elsevier B.V.This is an open access article under the CCBY-NC-ND license (http://creativecommons.org/licenses/by-nc-nd/4.0/)
Abstract
Tongue diagnosis can be an effective, noninvasive method to perform an auxiliary diagnosis any time anywhere, which can support the global need in the primary healthcare system. This work reviews the recent advances in tongue diagnosis, which is a significant constituent of traditional oriental medicinal technology, and explores the literature to evaluate the works done on the various aspects of computer- ized tongue diagnosis, namely preprocessing, tongue detection, segmentation, feature extraction, tongue analysis, especially in traditional Chinese medicine (TCM). In spite of huge volume of work done on auto- matic tongue diagnosis (ATD), there is a lack of adequate survey, especially to combine it with the current diagnosis trends. This paper studies the merits, capabilities, and associated research gaps in current works on ATD systems. After exploring the algorithms used in tongue diagnosis, the current trend and global requirements in health domain motivates us to propose a conceptual framework for the automated tongue diagnostic system on mobile enabled platform. This framework will be able to connect tongue diagnosis with the future point-of-care health system.
Keywords: Automated tongue diagnosis, Image processing, Machine learning, Mobile-enabled systems, Clinical decision support systems.
1. Introduction
The global demand for primary healthcare support and the advancement of technology enable the platforms for point-of-care (POC) diagnostics.1 Despite the recent advances in automated disease diagnosis tools, the requirement of blood serum via nonprofessionals, accuracy, reliability, detection time, and requirement of second confirmatory test are the challenges yet to overcome.2 Thus, temperature, tongue diagnosis, retinopathy, facial expressions, skin color, and surface could be few vital parameters for future smartphone-based clinical expert systems to attain simplicity, immediacy, noninvasiveness, and automatic analysis.
Tongue diagnosis is an effective noninvasive technique to evaluate the condition of a patient’s internal organ in oriental medicine, for example, traditional Chinese medicine (TCM), Japanese traditional herbal medicine, traditional Korean medicine (TKM).3, 4, 5 The diagnosis process relies on expert’s opinion based on visual inspection comprising color, substance, coating, form, and motion of the tongue.5, 6 Instead of tongue’s abnormal appearance and disease, traditional tongue diagnosis is more inclined to recognize the syndrome.7, 8, 9 For an example, the tongue coating white-greasy and yellow-dense appearance signifies cold syndrome and hot syndrome, respectively, which are tied with health conditions such as infection, inflammation, stress, immune, or endocrine disorders.10 These are two parallel, yet correlated syndromes in TCM. Eliminating the dependency on subjective and experience-based assessment of tongue diagnosis may greatly increase the scope for wider use of tongue diagnosis in the world including Western medicine. Computerized tongue inspection implicating light estimation, color correction, tongue segmentation, image analysis, geometry analysis, etc. could be an effective tool for disease diagnosis aiming to address these concerns.
Tongue diagnosis is not a widespread perception in western medical practice, which leads to one of the key challenges, that is, availability of the authentic classified dataset. On the other hand, Cheng et al11 questioned about the reliability and validity of practitioners of western medical system using TCM. They emphasized on the importance of the degree of agreement and criticized the adaptation of ‘proportion of agreement’. Belonging to oriental medicine, significant amount of work on tongue diagnosis is published in Chinese. To the authors’ best knowledge, the only detailed survey conducted up to 2012 was motivated by hardware and software of tongue diagnosis.5 There are recent advancement of mobile phone sensors, dynamics of its application, machine learning techniques, and expert systems,12, 13, 14, 15, 16, 17, 18 which has the potential to be implemented for automatic tongue diagnosis (ATD). Thus, there is a need to cover the insufficiency of focus on algorithms, machine learning techniques, and clinical decision support system (CDSS) related to tongue diagnosis. This work aims to investigate the scope of combining tongue diagnosis with the current research practice, making it work as an expert system without human intervention. The merits and capabilities of existing systems are explored to identify the research gap to achieve this goal. This work will provide an insight into the achievements so far and the mechanism to achieve the ultimate goal of automated tongue diagnosis.
Though few techniques used in case of tongue diagnosis find its applicability in different fields such as speech mechanism,19 tongue-operated system for Tetraplegia patients, this paper does not focus on such cases. This work considers the work published in English only. Manual, intended for digital diagnosis, semiautomatic, and ATD are considered to be reviewed. This work explores the state-of-the-art works done on tongue diagnosis and presents a direction toward a standard and complete work so that tongue diagnosis can be included as an auxiliary diagnosis to support the primary healthcare system. In Section 2, tongue diagnosis systems in general, especially hardware components associated with them are overviewed. In Section 3, tongue image segmentation and feature extraction techniques are critically reviewed. The inconsequential works done on specific disease detections are explored in later section (Section 4) followed by limited work done on mobile-enabled platform for tongue diagnosis (Section 5). The main focus of the work is algorithms used in different steps of ATD. On the basis of the research gap in the existing works, an overall conceptual diagram is presented in the last section (Section 6) to address the concerns undertaking the trend of the demand.
2. Tongue diagnosis systems
Because of advancement in image sensing modules, various image acquisition and analysis devices for tongue inspection commonly known as tongue diagnosis systems (TDSs) are surveyed and shaped. Jung et al5 reviewed both type of TDSs:
i) hardware consisting image sensing module, illumination module, compute, and
ii) control module and software for color correction, tongue image segmentation, and tongue classification in literature up to 2012.
To obtain quantitative and objective diagnostic results, the TDS needs to deliver reproducible tongue images under varying environmental conditions. In addition to commercially available digital cameras, many works used charge-coupled devices (CCD) with different configuration as the image sensing module.4, 6, 20, 21, 22, 23, 24, 25, 26, 27, 28 In recent literatures, both Cibin et al24 and Zhang and Zhang23 used 8-bit resolution 3-chip CCD cameras. The use of tongue image capturing device22, 23 is similar to the use of conventional digital camera. Kim et al,29 Zhang et al,21 and Jiang et al30 used CCD cameras to outline tongues, whereas Zhang et al22 placed D65 fluorescent tubes around the 8-bit resolution CCD camera to maintain uniform illumination. Different imaging schemes are described in Section 2.1. The work performed on color correction and light source estimation is explored in Section 2.2.
2.1. Different computerized tongue image analysis systems1
Specialized tongue capturing devices1 (e.g., tongue diagnostic information acquisition system) are often utilized to portray features such as tongue color, moisture, greasiness, indentation, pricking, fissure in tongue diagnostic information acquisition systems.3, 8, 10, 31 The CCD camera is light-sensitive, but it has the ability to produce quality images with less visual noise and distortion. When it comes to mobile phone camera, complementary metal-oxide-semiconductor (CMOS) is replaced by CCD due to power consumption and data-throughput speed. In order to improve the performance and gather more information, Zhi et al,32 Li and Liu,33 Yamamoto et al,6 and Li34 utilized hyperspectral imaging for tongue diagnosis. With an aim to attain the spectrum of an individual pixel in the image of a scene, the hyperspectral imaging is performed to find objects, identify materials, and detect processes. Though RGB is the most influential color model for tongue image analysis, to facilitate better distinguish between tongue coating and tongue body as well as differentiating among closely alike tongue and neighboring tissue colors, Zhi et al32 took the stance in favor of hyperspectral imaging. Unlike the traditional 2D imaging system with visible light waves, it can collect data from multiple bands. Zhi et al32 performed hyperspectral comparison of combinations of tissue types on 300 chronic Cholecystitis patients and 75 healthy subjects. Hyperspectral tongue images were analyzed through different classifiers. The performance of this approach was evaluated in contrast with the traditional RGB model as well. The authors stated their suggested method to be more successful than conventional RGB approach to a certain extent. Yamamoto et al6 used a hyperspectral camera to quantify color spectrum of both coated and uncoated tongue, lips, and periodical areas by considering highlight, shadow, and tongue coating.
Li34 captured hyperspectral tongue images at a series of wavelength to segment the tongue image, illustrated tongue colors and analyzed the tongue texture. A new algorithm was proposed using a Gabor filter for the tongue texture analysis. The Gabor filter is a linear filter, similar to human visual system. The two-dimensional Gabor filter is a Gaussian kernel function, which is modulated by a sinusoidal plane wave, useful for edge detection. The experimental result in Li34 showed promising for the postprocessing of computerized tongue diagnosis. Another example of a hyperspectral tongue imaging system is the work of Li et al,35 which presented a sublingual vein extraction algorithm using a hidden Morkov model. The sublingual vein is responsible for draining the tongue. These veins may be linked with number of diseases. Thus, the work involved outlining the spectral correlation and the multiband variability to accumulate more information about the tongue surface. Pixel-based sublingual vein segmentation (PBSVS) algorithm and spectral angle mapper (SAM) algorithm were used and the performance of their algorithms was analyzed based on 150 scenes of hyperspectral tongue images. Even in the presence of noise, the result was found to be more satisfactory than conventional algorithms. Li and Liu33 developed a pushbroom hyperspectral tongue imager to capture tongue images. They used SAM on 200 hyperspectral images to analyze tongue color based on the spectral response. The trajectory of tongue surface color distribution was tracked by the presented algorithm. For each color category, the correctness rate of color recognition was 85% and 88% for tongue substance and tongue coating, respectively. However, none of these works exercised disease classification exploiting the extracted features obtained from hyperspectral tongue images.
In order to attain three-dimensional geometry of a tongue, the 3D tongue diagnostic system could be an effective tool. Though 3D tongue imaging is more popular for visual speech synthesis,36 pronunciation recovery of impaired hearing individuals’, limited work37, 38, 39 has been done in the field of tongue diagnosis. TCM holds the view that the shape of the tongue can change and it indicates particular pathologies.40 In TCM, the shapes of tongues are classified into seven types: ellipse, square, rectangular, round, acute triangular, obtuse tri-angular, and hammer. The ellipse tongue represents the normal tongue.
An alternative view of tongue diagnosis is thus 3D imaging. The thickness measurement, impulsively changing the curvature of the tongue surface angle could be better represented with the 3D tongue modeling scheme. This is supported by Liu et al,39 who described a framework to reconstruct bending human tongue on the basis of multiview geometry. They used two light sources and a frame to fix the face-location. With the help of four cameras, they adjusted the exposure time and high speed frame rate. To locate the tongue, a laser device was utilized. The artificial features were created on a tongue surface through an adjustable laser beam. Finally, a finite-element-based representation of the tongue was attained.
Many of these devices, utilized by the existing systems, are capable of compensating lighting environmental condition (discussed in Section 2.2), but none of these systems is standalone (i.e., the system cannot provide the diagnosis or syndrome itself). Most systems are portable but they lack a collective intelligence to provide the final diagnosis.
2.2. Light source estimation and color correction
Lighting condition is considered to be an important prerequisite for tongue diagnosis. Different approaches have been taken to solve the challenging problem of lighting condition estimation. These solutions include controlled environment,27, 29 color correction,41 hardware based implementation, for example, tongue image acquisition device20 and ColorChecker.42 An image taken with the same camera may provide different tongue diagnosis due to the variation in the color properties at different lighting conditions. The imperfection in camera operation may cause some consequences.28 The inconsistence imaging with different devices may affect as an added disadvantage making it inconvenient to share or interchange.
Jang et al26 and Zhang et al20 used halogen tungsten lamp as the light source to capture tongue images. Both of them used low color temperatures. The color temperature of a lamp is the temperature of an ideal black-body radiator that radiates light of comparable hue to that of the lamp. It is a way to describe the light appearance provided by a light source, measured in degrees of Kelvin (K) on a scale from 1000 to 10,000. Typically, the light appearance of 2000–3000 K is referred to warm white, producing orange to yellow-white light; 3100–4500 K is cool or bright white emitting more neutral white light with probable blue tint and 4600–6500 K and above tries to imitate the daylight, producing a blue-white light and creating a crisp and invigorating ambience. However, the use of inadequate color temperature in the mentioned work failed to render color in high fidelity. Because of work done with reddish-biased images produced under halogen tungsten lamp, this is no longer a conventional method.
Research on tongue diagnosis includes placement of fluorescent lamp,25 standard light source installed in a dark chest,43 Köhler illumination,44 built-in LED lighting for tongue diagnosis. In general, Köhler illumination is used in optical microscopy as a specimen illumination method for transmitted and reflected light. It is useful to generate an incredibly even illumination of the sample. It has the ability to repress illumination source in the resulting image. However, the requirement of additional optical elements makes it more expensive. Another passing fad is taking images in public office environment.26 Hu et al41 analyzed tongue features observed under different lighting conditions such as fluorescent, incandescent, and halogen illuminant. They used Support Vector Machine (SVM) to make the system independent of lighting environment. To nullify inconsistent or varying lighting conditions, another current trend is hyperspectral imaging.33, 35 Another SVM-based work is recently reported to attain 94% accuracy to classify four tongue-related colors, that is, red, light red, and deep red.46 This work encompassed classification in two stages. The first stage involved the unsupervised machine learning, k-means. The background separation was partly involved at this stage. The later stage was based on SVM. The execution time was only 48 seconds, which shows the potential to be implemented as a real time tongue image segmentation technique. Because of the 2-layer segmentation process, SVM improved the color detection, that is, classification by 41.2%.
Even after using low color temperature halogen lamps, some work26 did not involve any color correction method. As a color correction scheme, the printed color card has been used in the earlier work.20, 25, 43, 45, 47 Some45, 47 used Munsell Color Checker as well. Munsell was considered to be the first color order system to separate hue, value, and chroma into perceptually uniform and independent dimensions and to systematically illustrate the colors in 3D space. Hu et al48 used SVM-based color correction matrix nullifying the dependency on controlled lighting environments and tongue positions. The lighting environment included with and without flashlights. They further improved their work and acquired better result.41 To the best of authors’ knowledge, this is the best work done so far in the field of tongue diagnosis for light estimation and color correction. Another popular convention is transforming the image into standard color space which could be achieved by both hardware and software manipulation.
On the other hand, recent advances of mobile phones are endeavoring to address lighting condition and color correction issue on its own. The camera lens, resolution, aperture, stabilizer, filter, multiframe imaging, and strong image processing software are able to manage the ambient condition better than ever. The back camera-front camera issue41 is also resolved for many phone brands. However, as chromatic features of tongue images hold the disease information, light estimation can still play a crucial role.
3. Image segmentation and feature extraction
The most challenging part of tongue diagnosis is an adequate segmentation and optimum feature extraction. Before starting the image segmentation, few works in literature preferred tongue detection and positioning. At the next stage, both color and geometric features need to be carefully extracted to feed into the subsequent steps.
3.1. Tongue detection and positioning
Zhong et al49 used mouth location method to locate dark hole’s position in the mouth and active appearance model (AAM) for automatic tongue segmentation. The method required a different initial contour for tongue body segmentation which made it technically infeasible.
In order to fix the tongue position, a guidance scheme for image acquisition system which outline the frontal and feedback gridlines, was sketched by Jung et al.4 The study included three categories, namely, nil, frontal, and frontal and profile gridline and 120 images were assessed based on profile angle and tongue area ratio. Jung et al4 discussed the relationship between diagnosis accuracy and repeatability of tongue features, for example, color and shape. The intraclass correlation coefficient was computed to examine repeatability. The result suggested an improvement in color and shape repeatability. Their study involved only healthy tongues which could be further used as a calibration model for related works.
3.2. Color models and chromatic features
The tongue diagnosis comprises recognizing the disease-related colors on the tongue surface and analyzing the texture. The tongue image segmentation steps such as edge detection and background–foreground separation are commonly processed in gray scale. However, the overall tongue image analysis needs to be processed in color format. The color gamut of a tongue image in different color spaces are portrayed in Fig. 1 as a point cloud. A summary of digital camera-based works in different color space focusing different features is presented in Table 1.
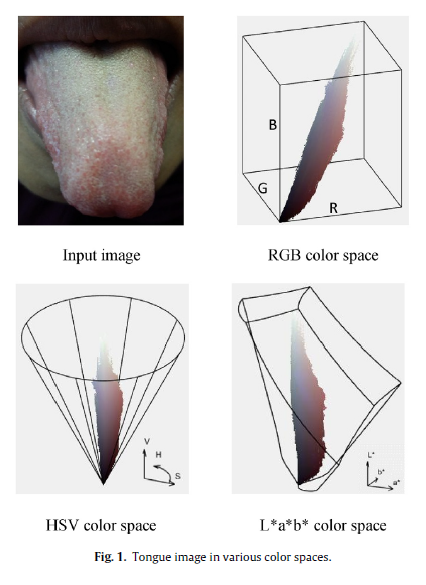
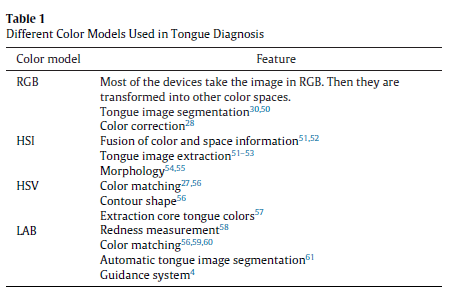
The RGB (Red, Green, Blue) is the most popular model for tongue diagnosis describing what kind of light needs to be emitted to produce a given color.30, 50, 62 The RGB space was utilized to extract tongue color features, for example, means and standard deviations of the colors per pixel within the region of interest.30 It is seen as true color in MATLAB, but the model is device dependent, which means there are many variations of RGB color space for the same image using different devices. HSI (Hue, Saturation, Intensity) and HSV (Hue, Saturation, Value or Brightness) are two cylindrical-coordinate representations of points in a RGB model. HSI is closer to human vision. Zhu et al51 favored HSI due to its specificity of the application in tongue image extraction. With the help of HSI color model, the greedy rules were utilized to develop the template. Then, the template was processed. After successful separation of background from the raw image, the template was found to be effective even for thick tongue coating. It can be useful for the quantitative evaluation of tongue.52 The hue and intensity were exploited by Jian-Qiang et al,55 for tongue image segmentation which worked well for regular tongue surfaces, but not for the irregular ones. Li et al56 used hue and brightness of HSV to decide the initial position of the tongue, whereas Jiang et al30 reported that HSV is unsuitable for tongue diagnosis as it has discontinuities in hue value around red, making it sensitive to noise. The color information is separated from the energy or image intensity in HSV, making the color space less noise-prone than in RGB. HSV being popular in the library like OpenCV for Android platform makes it a strong candidate to consider.
In the recently reported article on tongue diagnosis, researchers have found to prefer LAB over the other color space as it is more perceptually linear giving the advantage of effective visual inspection and comparison. L stands for lightness and ‘a’ and ‘b’ are the color opponent dimensions. Analyzing the tongue indices, the mean a* of the whole tongue area was found to be distinguishably different between people with and without Yin-deficiency.58 Thus, the color channel values can provide significant information about the patient’s condition.
Continuing with LAB color space, Jung et al4 explored diagnosis accuracy and considered the intraclass correlation coefficient to inspect tongue feature’s repeatability. The median value of tongue region, that is, tongue body was taken into account for color feature computation.63 Kawanabe et al59 examined tongue color, its body, and coating by analyzing tongue images of 1080 subjects. They cataloged tongue color and tongue coating into five and six categories, respectively in the L*a*b* color space. To compute tongue body and coating information, k-means clustering (an unsupervised machine learning technique) was employed. The authors suggested the outcome as a globally suitable tongue color diagnostic standard. However, the reliability was not quantified, especially for the tongue of unhealthy subjects. From the a* value difference, Kim et al58 separated the tongue coating from the tongue body area. The tongue coating was quantified by tongue coating percentage index. As the image was captured by a CTIS, the generated image needed to be transformed into L*a*b* from RGB.
Although tongue image capturing devices are useful, in literature, more works are done using a CCD camera. Hsu et al54 used a head mounted tongue capture device. Tongue image segmentation based on chromatic information is performed in the literature using all standard color models. However, sRGB (standard Red Green Blue) is found to be more popular choice to fuse color and space information.
Extracting primary core colors by an iterative method, Chen et al57 used Generalized Lloyd Algorithm (GLA) to obtain the color histogram. Because of the inadequacy of chromatic features, textural features were taken into account by an edge histogram descriptor. This ‘content-based image retrieval technology’ produced improved result, considering different weights for two features in retrieval. The work focused on feature extractions only, and disease classification was not considered. Inclusion of disease classification with same dataset of 268 images would have enlightened the readers more about the result obtained from feature extraction.
The artificial neural network (ANN) is not an exceptional choice for chromatic analysis. ANN has been successfully used in medical diagnosis due to its ability to process large volume of data, reduced probability of ignoring relevant information, and faster computational time.64 For example, the output of a wireless capsule endoscopy for evaluating the gastrointestinal tract is an 8 hours long video. To reduce the inconvenience of already over burden health system, Barbosa et al65 utilized an ANN to diagnose and classify small bowel tumors analyzing the visual information of wireless capsule endoscopy. An enhanced HSV color model was utilized for the segmentation purpose, using the convolutional neural network (NN).66 However, Cheung et al67 argued that the neural network is complex and time consuming. Table 2 demonstrates the practice of neural network for color-based analysis. However, Zhuo et al68 discouraged NN for online color correction in the current context due to the requirement of large sample size to train the NN-based mapping model. The polynomial-based correction method was favored for its lower computational complexity.
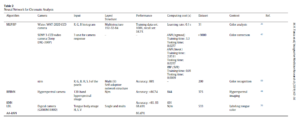
In the field of medical image processing, gray scale images are more prevalent than color images. The tongue diagnosis comprises recognizing the disease-related colors on the tongue surface and analyzes the texture. Therefore, one needs to address the issue of volume of colors to be managed. The complex part is the resemblance of the involved colors. Most of the disease-reflection features are reported to be located in the 2% region between the 100% and 98% of the full tongue color range.47 Thus, J measure based segmentation (JSEG) algorithm is performed to test the homogeneity of a given color-texture pattern. The method involved unsupervised machine learning techniques such as k-means clustering and a hierarchical clustering (called agglomerative clustering). This segmentation method is computationally more feasible than model parameter estimation. The method proposed by Deng and Manjunath71 is illustrated in Fig. 2. The color quantization starts with the peer group filtering,72 which provides a smooth image by removing the noise. It also provides a smoothness indicator value. The weight to each pixel is assigned in such a way that pixel at textured area’s weight is less than smooth area’s weight. The implementation of agglomerative clustering algorithm increases the pixel of approximate same color, decreasing the global distribution. The color class-map is applied to local window and then J-image is obtained using Fisher’s multiclass linear discrimination.
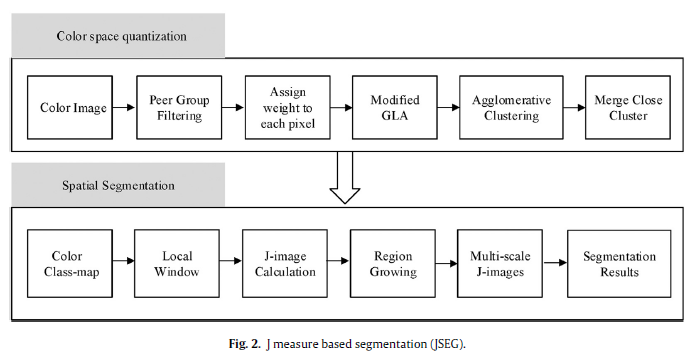
The implementation of JSEG, proposed by Deng and Manjunath,71 is shown in Fig. 3 to illustrate the effective color reduction keeping the useful information intact.
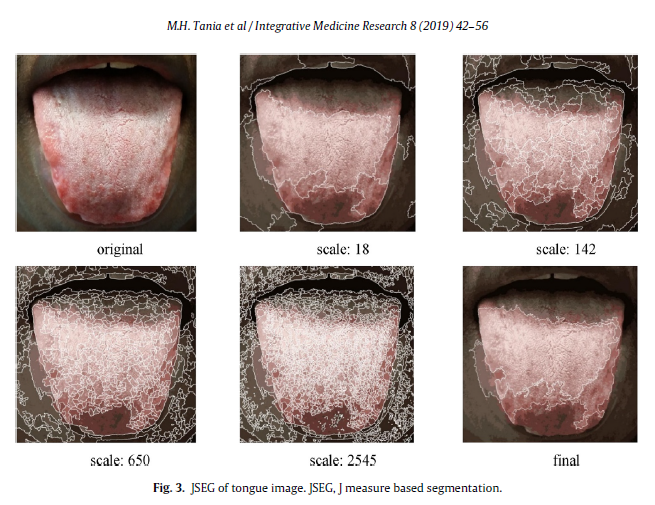
Although chromatic features are essential to analyze, it is not sufficient enough to provide the complete information. Thus, this work will continue to discuss about different image processing techniques in the next section.
3.3. Edge detection techniques
Edge detectors are essential tools for tongue diagnosis and one of the most popular techniques. The prewitt, sobel, and Laplacian filters are commonly used edge detectors for medical image processing. To separate the tongue from face and mouth (i.e., background–foreground separation), different versions of canny filter73 are used21, 56, 74, 75. Shi et al75 used canny to find the tongue tip point. Zhang et al21 used CCD camera to outline tongue via canny algorithm and then utilized feature extraction to diagnose diseases. The authors transformed the image into gray scale and an optimized canny algorithm to obtain the edge image. Kanawong and Xu76 presented a complete automatic tongue detection and tongue segmentation framework method excluding any parameter adjustment and initialization. They proposed a Principal Component Analysis (PCA)-based tongue detection algorithm and hybrid tongue segmentation utilizing mean shift algorithm, canny edge detection algorithm, and Tensor Voting algorithm. However, the use of only one sample makes the system incompetent. The Sobel is another popular filter to be found in the literature.56, 59 Few works have been done using mathematical morphology. Li et al56 compared different tongue image extraction methods such as geodesic active contour, HSI color model (mathematical morphology and sequential algorithm), and presented a method that involves multiobjective greedy rules to employ fusion of color and space information. Jian-Qiang et al55 utilized morphological opening to eliminate the thin link of lower lip attached with the tongue image and morphological closing to get rid of the small holes on tongue.
3.4. Contour shape
The contour detection is quite prevalent for tongue region segmentation. The snake model finds its usefulness on tongue image segmentation with modifications.50, 75, 77, 78, 79 The dual snake was used for tongue segmentation with 92.89% accuracy.78 Noise removal and HIS color model transformation were conducted before implementing the algorithm to achieve an accurate contour. However, the method was not feasible due to the hurdle to attain the initial contour, both inside and outside. Shi et al79 used snake geodesic active contour (GAC) model for tongue image segmentation. GAC is also known as geometric active contour or conformal active contours. The model is inspired by the Euclidean curve shortening evolution. On the basis of the object of the image, the contour splits and merges. Shi et al79 categorized the images of the database by tongue colors and it contained four classes and ten branches. The double geodesic flow was applied after dividing the tongue image into two parts based on previous knowledge of tongue shape and location. The result showed low error and good true positive volume fraction (98%) compared to other works. But because of expensive computational time, it is not feasible for real time solution. Moreover, the system is not full-proof for background separation in case of irregular tongue images. Later, the work continued with color control-geometric and gradient flow snake (C2G2FSnake) algorithm for fully automated tongue segmentation, keeping the number of classifications and branches same.75 The dataset included 30 images. This method enhanced the curve velocity. Though the work showed good accuracy and less complexity, it needed four points to be specified for initial contour formation. Chen et al61 performed tongue image segmentation utilizing the same dataset via MATLAB and the test result showed 98.4% accuracy taking 11.46 seconds to compute with C2G2FSnake. Testing on 40 images, recent work has been reported to achieve 75% accuracy.80
3.5. Watershed transformation
The word watershed signifies a ridge that divides areas drained by different river systems. The geographical area draining into a water source, for example, river or reservoir is called the catchment basin. The watershed algorithm is adaptable to various kinds of images, for example, blood cells, stars, toner spots on a printed page, DNA microarray elements. This segmentation algorithm is capable to differentiate incredibly complex images. However, the performance depends on correct choice of markers to avoid oversegmentation. The watershed transform is also utilized as a segmentation technique for tongue diagnosis.50, 61, 81
A possible route for watershed transform on tongue could be contrast-limited adaptive histogram equalization (after preprocessing), thresholding, filtering, and then overlaying the perimeter as shown in Fig. 4. The last image of Fig. 4 is where watershed algorithm was employed in this work and this was introduced by Eddins82 for cell segmentation with microscopic images. The image does not contain the anticipated textural information. The region of interest is cropped and the rest of the image information is not useful.
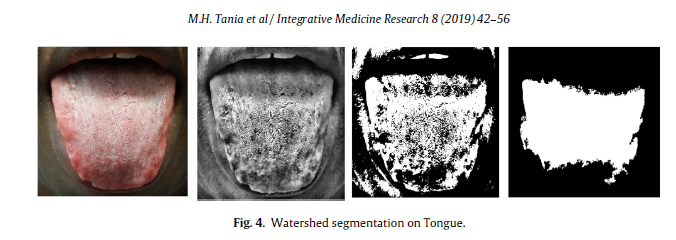
Snake models are often combined with watershed transform to produce better edge detection. Wu et al81 used such combination on 120 images, where the initial contour was generated using watershed transform whereas the active contour model was used to converge to the exact edge. However, there may not be a clear edge border for tongue images in natural setting like the ones used in this work. Taking RGB tongue images as input, Ning et al50 sequentially used gradient vector flow (GVF), watershed transformation, and then an interactive region merging method called maximal similarity based region merging (MSRM) and finally snake model for refinement. The idea was to avert oversegmentation of watershed as GVF can well preserve main edge structures while removing trivial structures.
3.6. Tongue shape based analysis
In order to analyze the shape of the tongue, one may require contour extraction and tongue shape correction. Zhang and Zhang23 used decision tree to classify five different types of tongue shapes among 130 healthy and 542 diseased people. They included 13 geometric features. Their work outline is sketched in Fig. 5.
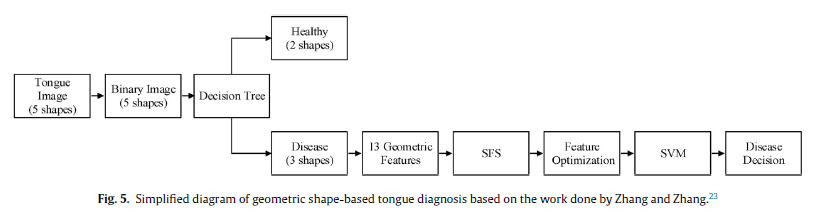
Huang et al40 corrected the tongue deflection based on three geometric categories and analyzed tongue shape based on seven geometric features. The Analytic Hierarchy Process (AHP) was utilized for tongue classification by computing relative influences of different parameters such as length, area, and angle factor. Each AHP module represented each tongue shape class. The ambiguity and impression between the quantitative features and the shape classes were presented by the fuzzy fusion framework. This framework combined seven AHP modules. The study included 362 tongue samples and the result showed success to decrease tongue shape deflection. The classification accuracy was 90.3%. The authors compared the result with kNN83 and linear discriminant analysis,99 where the accuracy was 78.2% and 82.9%, respectively. Although their study includes unhealthy tongues, the correlations between disease types and tongue shapes are not taken into account.
4. Disease classification
Tongue diagnosis deals with syndrome, rather than disease itself. Xu et al84 classified syndromes using machine learning techniques. Another syndrome classification-based work, conducted by Zhao et al,85 focused on searching for its correlation with viral hepatitis. Kim et al58 utilized statistical analysis to prove that the self-reported Yin deficient patients may have more redness in tongue and less tongue coating in comparison to non-Yin-deficient control group. Statistical analysis was further utilized to determine the relationship between ischemic stroke with and without retroflex tongue among 308 patients.86 A statistical analysis was utilized by Kim et al87 to track the treatment of functional dyspepsia. This work analyzed the change in the Nepean Dyspepsia Index scores to indicate the primary outcome. The baseline and the images taken after four weeks were examined to achieve this goal. More parameters (such as tongue coating thickness) were added to find out a few secondary outcomes.
Effective CDSS may be yet to be established, but there is no denial that CDSS has the potential to improve healthcare by linking health observations with health knowledge to influence health choices by clinicians. The diagnosis decision support systems (DDSS) is a specialized CDSS for diagnosis that requests for the patients data, for example, questionnaires, images, previous medical record. In response to the request, the system provides a disease decision or proposes a set of appropriate diagnoses. DDSS are becoming popular day by day and is being tested in dynamic scenario.88, 89
Zhang et al20 presented a Bayesian network classifier-based system to diagnose pulmonary heart disease, appendicitis, gastritis, pancreatitis, and bronchitis with about 75% accuracy. Their system works in real-time to point out quantitative features from tongue images. They used Support Vector Regression (SVR) for color correction and Bayesian classifier for chromatic and textural measuring. Their work included 544 patients and 56 healthy subjects. The diagnosis accuracy was found to be 100% for the healthy class. The result was not promising for diseases such as nephritis, gastroduodenal perforation, and abdominal tuberculosis. Though accuracy for Leukemia was only 67.6% and the sample size was 37, more attention can be drawn toward such cancer diagnosis.
Abe et al90 tried to establish the relationship between tongue coating and aspiration pneumonia. The tongue plaque index (TPI) was utilized to investigate the association of selected oral bacteria present in saliva and tongue-coating. The subjects were 71 edentulous elderly aged over 65 years. The outcome suggested that tongue-coating can be used as a risk indicator for edentate (incisor and canine toothless) subjects to detect aspiration pneumonia.
Han et al31 investigated tongue coating images as a probable tool for a colorectal cancer diagnosis. They appraised tongue images captured by a specialized image acquisition device to study the impact on coating thickness in the presence of responsible microbial particle. The result showed clear variation between microbial community structures in case of patients suffering from colorectal cancer. The presented analysis indicates that tongue coating analysis may be a novel potential biomarker for colorectal cancer diagnosis. Han et al8 further extended their study with improved sample size for early diagnosis of different types of cancer. Their study showed that there was a color variation between healthy and cancer tongues, one was reddish while the other was purple respectively. The cancer patients’ tongues lack in relative abundance of bacterial microorganisms such as Neisseria, Haemophilus, Fusobacterium, and Porphyromonas. The work indicated toward a possible cancer screening and early diagnosis method through tongue and tongue coating analysis.
Zhang et al22 used tongue color gamut on 1045 images to differentiate between healthy tongue and tongue with disease and to diagnose different diseases including chronic kidney disease, diabetes nephritis, hypertension, verrucous and erosive gastritis, pneumonia, nephritic syndrome, chronic cerebral circulation insufficiency, upper respiratory tract infection, coronary heart disease, chronic bronchitis, and mixed hemorrhoid. They used k-NN and SVM to find whether the subject is healthy or not. Both of these techniques provided 91.99% classification accuracy. Later on, clustering was performed on 11 diseases with 70% accuracy. Though SVM and k-NN both show similar outcome for clustering, SVM performs better. For both cases, diabetes and hypertension classification result are poor.
Zhang and Zhang23 conducted quantitative analysis on 672 images to distinguish between healthy and diseased tongue and to diagnose diabetes mellitus, different gastritis, nephritis, and coronary heart disease. Samples were collected from Traditional Chinese Medicine Hospital but the images were classified based on western medical practice. The decision tree was used to classify among five different types of tongue shapes, SVM was used to classify diseases for each tongue shape utilizing 13 geometric features, and number of features were optimized by Sequential Forward Selection (SFS). They also used k-NN but it gave poor result. The average accuracy of disease classification is 76.24%. In addition to tongue shape and geometric features, fusion of other features such as tongue color, fur, and fissure could be explored for better diagnosis of the diseases.
With an aim to diagnose diabetes, tongue image information of total 827 subjects, both diabetic and non-diabetic obtained from TDA-1 digital tongue instrument were analyzed.91 Tongue body and coating separation, color, and textural features were examined by division merging, chrominance-threshold, SVM, and few other algorithms. After sample equalization and feature normalization, 23 input parameters including information of the subject, tongue color, and texture PCA were used to reduce the dimensions of the feature. Optimizing the kernel parameter by GA, SVM showed 78.77% accuracy for diabetes prediction. Though the prediction accuracy is low, the work carries clear indication about the potential of tongue diagnosis for diabetes detection. In future, inclusion of other tongue features may increase the accuracy.
Inspired by deep convolutional neural network (CNN), a feature extraction technique named constrained high dispersal neural networks was implemented on tongue images of 315 people.92 The objective of this feature extraction was to separate healthy and unhealthy class. A weighted LIBLINEAR SVM was trained to perform the task, which showed 91.14% accuracy. The authors claimed the system to be faster than CNN; however, to be implemented in real-time, one has to be careful about using deep neural network, especially when the accuracy is still low and the system cannot provide decision on specific disease.
In recent years, researchers have been working on the ATD. But most of them are concerned only up to feature extraction stage. On the other hand, CDSS-based works paid an inadequate attention on the prior steps involved, which raises the issue of compatible standardization. Besides, the limited work done on tongue diagnosis-based disease detection is not on the same diseases. Thus, it becomes hard to compare the accuracy and repeatability of the best decision support system found in this field. Moreover, the reported works suggest the accuracy slightly under 80%, which may not be acceptable in many cases of CDSSs.
There are few works93 done on early stage breast cancer (BC) detection. Nine tongue features of both breast cancer patient (stage 0 and 1) and healthy persons were investigated in a hope to diagnose BC at an early stage to ensure timely treatment to increase the likelihood of recovery, lowering the relapse rate. The considered features are tongue color, quality, fur, fissure, red dot, ecchymosis, tooth mark, saliva, and tongue shape. The subdivision of the extracted features according to the corresponding organs such as spleen–stomach, liver–gall-left, liver–gall-right, kidney, and heart–lung area are illustrated in Fig. 6.
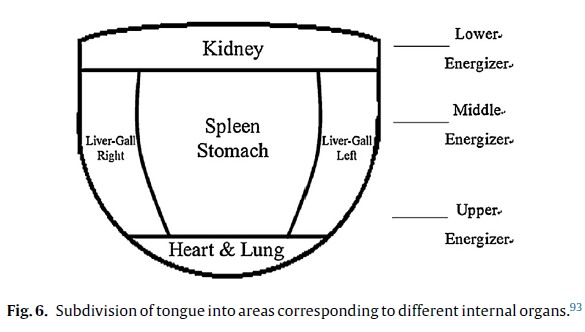
The data of 57 early-stage BC patients and 60 healthy people were utilized for training, whereas the data of 10 early-stage BC patients and 10 healthy people were applied for testing. The tongue features along with Mann–Whitney test were exploited to perform the logistic regression. Though the result is not good for the early stage or potential cancer patient, more feature extraction techniques as well as different features could be explored to attain better accuracy. The accuracy for healthy tongue was varied from 80% to 90%. Higher accuracy was attained using lesser number of features. The work was further upgraded without any significant change in the result.93
A summary of algorithms used in disease diagnosis is provided in Table 3.
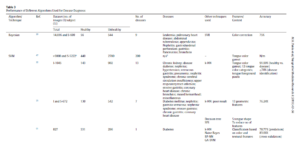
5. Mobile-enabled tongue diagnosis schemes
In 2009, American Recovery and Reinvestment Act encouraged widespread adoption of health information technology (HIT) via Health Information Technology for Economic and Clinical Health Act (HITECH). Gradually these HITs are becoming more robust and diverse. Disease diagnosis on a mobile platform has gained the attention of researchers and developers in the last few years.95
Though available smartphone technology offers enormous prospect to employ tongue diagnosis for disease classification, very few work has been done exploiting existing means. To authors’ best knowledge, only one mobile application is available in iOS and android platform called i-Tongue © University of Missouri.96 Because of openness, total interface customization, universality, security, few more tongue diagnosis applications are initiated focusing android platform than iOS.21, 41, 48
The research work conducted by Otsu97 and Hsu et al54 was taken under consideration by Hu et al,41 for tongue region segmentation and tongue diagnosis, and the algorithm was tested in smartphones such as Samsung Galaxy S2 and S3, HTC Sensation, and J Z32 providing similar outputs and server running in PC is able to perform analysis of each image taking below 3 seconds. Hu et al pointed out that most of the mobile phone camera other than tongue with any kind of deformity this work is promising for fur and fissure detection. Though based on this work’s output, the team is working on liver disease diagnosis, and this work does not include any decision support system and thus predominantly relies on a physician’s opinion. Zhang et al21 used CCD camera to outline tongue via canny algorithm and then utilized feature extraction to diagnose diseases.
Taking teeth color as a standard, Ini98 presented a smartphone-based tongue color calibration method. 20 female subjects were chosen for the study, continued for a month. K-means algorithm was used to detect tongue coating differentiating from other body parts. However, the distinguishing method itself was unfitting to start with.
Taking lighting condition into account, Hu et al48 used a smartphone to calculate SVM-based color correction matrix and to automatically detect fur and fissure of tongue. The lighting environment included with and without flashlights. This work focused on detection accuracy, but robustness was not explored.
Like most diagnosis-based health and fitness mobile applications, iTongue © University of Missouri96 is available for both guest and registered users in google play and apple app store. The height (cm) and weight (kg) of the user has to be provided during registration. There is a series of questions users must provide answer to; otherwise no result will be generated. There are some additional questions for registered users; few of them are gender-specific. Using these answers, the application generates the result and recommendations including constitute of qi asthenia, yang asthenia, yin asthenia, phlegm-dampness, and damp-heat. Each constitute can recommend environment, exercise, emotion, and diet. The guest version cannot store any images. The application removes the image when the user tries to take another picture. There is (x, y) axis to guide the user about tongue exposure in front of the phone camera. The registered users can save the history and use stored images from the phone. Image segmentation, feature extraction, and machine learning techniques are used to process the result. The user can request for manual assessment by TCM professionals with a fee. The current version 2.0.2 is an update released on Sep 14, 2016 requires minimum android 2.2 or iOS 5.1.1 and can be operated in English, Simplified Chinese, and Traditional Chinese. The update includes sharing features as well. The application is intended for an auxiliary diagnosis with questionable accuracy and reliability.
A mobile enabled system for tongue diagnosis without any human intervention is outlined in Tania et al2 (Fig. 7). Such a system would comprise image processing and machine learning algorithms to act as a standalone system. However, the implementation on mobile platform was not included in the paper.
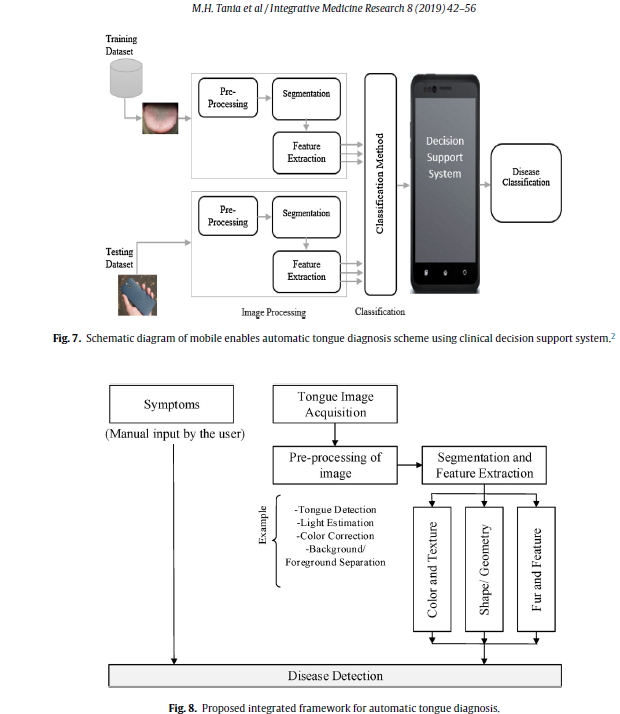
6. Proposed framework and discussion
On the basis of our study, we have identified the research gaps to be addressed to develop an intelligent tongue diagnosis system. They are summarized as below.
- Unlike the western research convention, it is not a common practice to deposit the classified authentic tongue images in an open access database, thereby limiting the scope of improvement. There should be benchmark datasets of classified tongue images in the open access database to broaden the horizon of tongue diagnosis. Without a benchmark dataset, it is hard to perform comparable experiments, verify, validate, or improve the results.
- There is a lack of overall consistency in the ATD systems including characteristics of the input image, for example, image quality, design guideline, image segmentation, and feature extraction. Thus, the accuracy, robustness, and reliability are inevitably improbable.
- There is an infrequency of interchangeable scheme, making it incomprehensible to utilize the best found result from the prior step as an input for the subsequent step for different work.
- To the best of our knowledge, there is no complete work (i.e., starting from the light estimation to CDSS) that has been conducted in the literature.
The tongue diagnosis requires more autonomy to reach its full potential and ensure its widespread application in the digital health system. On the basis of the work done in the literature, a conceptual diagram is illustrated in Fig. 8 as a completely ATD system using CDSS, independent of human expert involvement. The idea is to incorporate disease detection with extracted features from tongue diagnosis in a mobile enabled platform (Fig. 7).
The commercial health applications, for example, iPhone health app usually provides provision for manual input regarding symptoms. The manual input of disease symptoms can be based on selected diseases. Any of the standard questionnaire set, for example, TCM, Kampo can be utilized. A polythetic approach on the mobile platform should consider the symptoms provided by the user with the color, texture, and geometric features.
With the improvement of hardware, preprocessing requirements are reducing day by day. The commercially available mobile device possesses camera resolution as high as 12–16 megapixel, with F1.7 lens. Higher focus and built-in automatic adjustment features are narrowing down, and the necessity of light estimation and color correction is abridging or nullifying. Moreover, a strong image processing algorithm can often manage the lighting condition by itself. The extracted features (e.g., fur, texture, shape), subsequent to image segmentation, can be utilized for classification. All these information, including the symptoms, need to be fused together by a fusion algorithm to provide the final result. The conceptual diagram can be trained offline and the whole diagnosis system can be deployed as a smartphone application to avail the system as a homecare or mobile setting (Fig. 7).
7. Conclusion
This paper explored the standardized and automated tongue diagnosis systems to demonstrate its potential. The investigation is in favor of tongue diagnosis to make it an integrated part of auxiliary diagnosis system within the primary healthcare system and an early diagnostic tool. Because the chromatic, textural and geometric shape based analysis of tongue projects indispensable information about health status. Remarkable work has already been done on different steps, for example, tongue image segmentation, background–foreground separation, healthy–unhealthy classification, and few specific disease-related works. This work explored each of these steps in the literature, especially focusing on the algorithms. Identifying some general trends, this section broadly summarizes the research gap.
While evaluating the prospect of tongue diagnosis, the absence of CDSS was noticeable. Our study indicated that the ATD embracing a CDSS can open the door for standalone systems, rather than relying on limited TCM experts only. The recent advancement in the field of camera of smart devices, classifiers, and CDSS algorithms present the opportunity to develop a real-time framework and standard protocol for everyone to be followed so that collective knowledge can be built on existing one. However, it was also noticeable from our survey that the transition from one step to another is incomprehensible due to ‘casual’ diversity in practice. Thus, the need for standardization is inevitable from the literature, as everyone is defining their own system for various steps but in most cases, there is no way to put them together. To proceed with the research on tongue diagnosis, undeniably there is a need for an open access authentic dataset with classified images of tongue.
The volume of work done in the literature does not combine the overall system to make it human intervention-free. The literature reflected the lack of a complete standalone system from capturing the tongue picture to attain the disease diagnosis. On the basis of the literature review, this work proposed an integrated framework to develop such a system. The derivation of general recommendations may help those who intend to bring tongue diagnosis into modern technology enabled practice.
Acknowledgements
The research work has been funded by Erasmus Mundus Partnerships Action 2 “FUSION” (Featured eUrope and South asIa mObility Network) project of Erasmus Mundus. Grant reference number: 2013-3254 1/001001.
References
1. Contreras-naranjo J.C., Wei Q., Ozcan A. Mobile phone-based microscopy, sensing, and diagnostics. IEEE J Sel Top Quantum Electron. 2016;22:1–14. [Google Scholar]
2. Tania M.H., Lwin K.T., Hossain M.A. 2016 10th International Conference on Software, Knowledge, Information Management & Applications (SKIMA). Chengdu: IEEE. 2016. Computational complexity of image processing algorithms for an intelligent mobile enabled tongue diagnosis scheme; pp. 29–36. [Google Scholar]
3. Kim J., Han G., Ko S.J., Nam D.H., Park J.W., Ryu B. Tongue diagnosis system for quantitative assessment of tongue coating in patients with functional dyspepsia: a clinical trial. J Ethnopharmacol. 2014;155:709–713. [PubMed] [Google Scholar]
4. Jung C.J., Kim K.H., Jeon Y.J., Kim J. Improving color and shape repeatability of tongue images for diagnosis by using feedback gridlines. Eur J Integr Med. 2014;6:328–336. [Google Scholar]
5. Jung C.J., Jeon Y.J., Kim J.Y., Kim K.H. Review on the current trends in tongue diagnosis systems. Integr Med Res. 2012;1:13–20. [PMC free article] [PubMed] [Google Scholar]
6. Yamamoto S., Tsumura N., Nakaguchi T., Namiki T., Kasahara Y., Terasawa K. Regional image analysis of the tongue color spectrum. Int J Comput Assist Radiol Surg. 2011;6:143–152. [PubMed] [Google Scholar]
7. Su W., Xu Z.Y., Wang Z.Q., Xu J.T. Objectified study on tongue images of patients with lung cancer of different syndromes. Chin J Integr Med. 2011;17:272–276. [PubMed] [Google Scholar]
8. Han S., Yang X., Qi Q. Potential screening and early diagnosis method for cancer: tongue diagnosis. Int J Oncol. 2016;48:2257–2264. [PMC free article] [PubMed] [Google Scholar]
9. Pang B., Zhang D., Li N., Wang K. Computerized tongue diagnosis based on Bayesian networks. IEEE Trans Biomed Eng. 2004;51:1803–1810. [PubMed] [Google Scholar]
10. Jiang B., Liang X., Chen Y., Ma T., Liu L., Li J. Integrating next-generation sequencing and traditional tongue diagnosis to determine tongue coating microbiome. Sci Rep. 2012;2:936. [PMC free article] [PubMed] [Google Scholar]
11. Cheng T.L., Lo L.C., Huang Y.C., Chen Y.L., Wang J.T. Analysis of agreement on traditional Chinese medical diagnostics for many practitioners. Evid-based Complem Altern Med. 2012;2012 [PMC free article] [PubMed] [Google Scholar]
12. Reddington A.P., Trueb J.T., Freedman D.S., Tuysuzoglu A., Daaboul G.G., Lopez C.A. An interferometric reflectance imaging sensor for point of care viral diagnostics. IEEE Trans Biomed Eng. 2013;60:3276–3283. [PMC free article] [PubMed] [Google Scholar]
13. Switz N.A., D’Ambrosio M.V., Fletcher D.A. Low-cost mobile phone microscopy with a reversed mobile phone camera lens. PLOS ONE. 2014;9:e95330. [PMC free article] [PubMed] [Google Scholar]
14. Skandarajah A., Reber C.D., Switz N.A., Fletcher D.A. Quantitative imaging with a mobile phone microscope. PLOS ONE. 2014;9:e96906. [PMC free article] [PubMed] [Google Scholar]
15. Smith Z.J., Chu K., Espenson A.R., Rahimzadeh M., Gryshuk A., Molinaro M. Cell-phone-based platform for biomedical device development and education applications. PLOS ONE. 2011;6:e17150. [PMC free article] [PubMed] [Google Scholar]
16. Bogoch I.I., Andrews J.R., Speich B., Utzinger J., Ame S.M., Ali S.M. Mobile phone microscopy for the diagnosis of soil-transmitted helminth infections: a proof-of-concept study. Am J Trop Med Hyg. 2013;88:626–629. [PMC free article] [PubMed] [Google Scholar]
17. Petersen C., Chen T., Ansermino J., Dumont G. Design and evaluation of a low-cost smartphone pulse oximeter. Sensors. 2013;13:16882–16893. [PMC free article] [PubMed] [Google Scholar]
18. Daaboul G.G., Lopez C.A., Yurt A., Goldberg B.B., Connor J.H., Ünlü M.S. Label-free optical biosensors for virus detection and characterization. IEEE J Sel Topics Quant Electron. 2012 [Google Scholar]
19. Lu J., Yang Z., Okkelberg K., Ghovanloo M. Joint magnetic calibration and localization based on expectation maximization for tongue tracking. IEEE Trans Biomed Eng. 2017;65:52–63. [PMC free article] [PubMed] [Google Scholar]
20. Zhang H.Z., Wang K.Q., Zhang D., Pang B., Huang B. Computer aided tongue diagnosis system. 2005 IEEE Engineering in Medicine and Biology 27th Annual Conference, vol. 7; Shanghai; 2005. pp. 6754–6757. [PubMed] [Google Scholar]
21. Zhang Q., Shang H.L., Zhu J.J., Jin M.M., Wang W.X., Kong Q.S. A new tongue diagnosis application on android platform. Proceedings – 2013 IEEE International Conference on Bioinformatics and Biomedicine, IEEE BIBM 2013; Shanghai; 2013. pp. 324–327. [Google Scholar]
22. Zhang B., Wang X., You J., Zhang D. Tongue color analysis for medical application. Evid-based Complem Alternat Med. 2013;2013:264742. [PMC free article] [PubMed] [Google Scholar]
23. Zhang B., Zhang H. Significant geometry features in tongue image analysis. Evid-based Complem Alternat Med. 2015;2015:1–8. [PMC free article] [PubMed] [Google Scholar]
24. Cibin N.V., Franklin S.W., Nadu T. Diagnosis of diabetes mellitus and NPDR in diabetic patient from tongue images using LCA classifier. Int J Adv Res Trends Eng Technol. 2015;II:57–62. [Google Scholar]
25. Chiu C.C. A novel approach based on computerized image analysis for traditional Chinese medical diagnosis of the tongue. Comput Methods Programs Biomed. 2000;61:77–89. [PubMed] [Google Scholar]
26. Jang J.H., Kim J.E., Park K.M., Park S.O., Chang Y.S., Kim B.Y. Proc Second Jt 24th Annu Conf Annu Fall Meet Biomed Eng Soc [Engineering Med Biol.], vol. 2. 2002. Development of the digital tongue inspection system with image analysis; pp. 1033–1034. [Google Scholar]
27. Li C.H., Yuen P.C. Tongue image matching using color content. Pattern Recognit. 2002;35:407–419. [Google Scholar]
28. Wang X., Zhang D. An optimized tongue image color correction scheme. IEEE Trans Inf Technol Biomed. 2010;14:1355–1364. http://ieeexplore.ieee.org/stamp/stamp.jsp?tp=&arnumber=5570961 [PubMed] [Google Scholar]
29. Kim M., Cobbin D., Zaslawski C. Traditional Chinese medicine tongue inspection: an examination of the inter- and intrapractitioner reliability for specific tongue characteristics. J Altern Complem Med. 2008;14:527–536. [PubMed] [Google Scholar]
30. Jiang L., Xu W., Chen J. Digital imaging system for physiological analysis by tongue colour inspection. 2008 3rd IEEE Conference on Industrial Electronics and Applications; Singapore; 2008. pp. 1833–1836. [Google Scholar]
31. Han S., Chen Y., Hu J., Ji Z. Tongue images and tongue coating microbiome in patients with colorectal cancer. Microb Pathog. 2014;77:1–6. [PubMed] [Google Scholar]
32. Zhi L., Zhang D., Yan J.qi, Li Q.L., Tang Q.lin. Classification of hyperspectral medical tongue images for tongue diagnosis. Comput Med Imaging Graph. 2007;31:672–678. [PubMed] [Google Scholar]
33. Li Q., Liu Z. Tongue color analysis and discrimination based on hyperspectral images. Comput Med Imaging Graph. 2009;33:217–221. [PubMed] [Google Scholar]
34. Li Q. 2008 2nd Int Conf Bioinforma Biomed Eng. 2008. Hyperspectral imaging technology used in tongue diagnosis; pp. 2579–2581. [Google Scholar]
35. Li Q., Wang Y., Liu H., Guan Y., Xu L. Sublingual vein extraction algorithm based on hyperspectral tongue imaging technology. Comput Med Imaging Graph. 2011;35:179–185. [PubMed] [Google Scholar]
36. Jiang C., Luo C., Yu J., Li R., Wang Z. 2014 IEEE Int Conf Multimed Expo Work, ICMEW. 2014. Modeling a realistic 3D physiological tongue for visual speech synthesis; pp. 2–7. [Google Scholar]
37. Jian Z., Lijuan S., Lirong W., Qinsheng D., Yajuan S. 2012 Fifth Int Conf Intell Networks Intell Syst. 2012. Dynamic extraction method of 3D parameters of tongue for pronunciation recovery about impaired hearing children; pp. 340–343. [Google Scholar]
38. Lv H., Cai Y., Guo S. Int Conf Signal Process Proceedings, ICSP, vol. 3. 2012. 3D reconstruction of tongue surface based on photometric stereo; pp. 1668–1671. [Google Scholar]
39. Liu Z., Wang H., Xu H., Song S. 3D tongue reconstruction based on multi-view images and finite element. Adv Inf Sci Serv Sci. 2011;3 [Google Scholar]
40. Huang B., Wu J., Zhang D., Li N. Tongue shape classification by geometric features. Inf Sci (NY) 2010;180:312–324. [Google Scholar]
41. Hu M.-C., Cheng M.-H., Lan K.-C. Color correction parameter estimation on the smartphone and its application to automatic tongue diagnosis. J Med Syst. 2016;40:18. [PubMed] [Google Scholar]
42. McCamy C., Marcus H., Davidson J. A color-rendition chart. J Appl Photog Eng. 1976;2:95–99. [Google Scholar]
43. Wang Y., Zhou Y., Yang J., Xu Q. An image analysis system for tongue diagnosis in traditional Chinese medicine. In: Zhang J., He J.-H., Fu Y., editors. Computational and Information Science: First International Symposium, CIS 2004, Shanghai, China, December 16–18, 2004. Proceedings. Springer Berlin Heidelberg; Berlin, Heidelberg: 2005. p. 1181-1186. [Google Scholar]
44. Liu Z., Li Q., Yan J., Tang Q. A novel hyperspectral medical sensor for tongue diagnosis. Sens Rev. 2007;27:57–60. [Google Scholar]
45. Cai Y.C.Y. IMTC/2002 Proc 19th IEEE Instrum Meas Technol Conf (IEEE Cat No00CH37276), vol. 1. 2002. A novel imaging system for tongue inspection; pp. 21–23. [Google Scholar]
46. Kamarudin NDi, Ooi C.Y., Kawanabe T., Odaguchi H., Kobayashi F. A fast SVM-based tongue’s colour classification aided by k-means clustering identifiers and colour attributes as computer-assisted tool for tongue diagnosis. J Healthc Eng. 2017;2017 [PMC free article] [PubMed] [Google Scholar]
47. Wang X., Zhang B., Yang Z., Wang H., Zhang D. Statistical analysis of tongue images for feature extraction and diagnostics. IEEE Trans Image Process. 2013;22:5336–5347. [PubMed] [Google Scholar]
48. Hu M.-C., Zheng G.-Y., Chen Y.-T., Lan K.-C. Automatic tongue diagnosis using a smart phone. 2014 IEEE International Conference on Systems, Man, and Cybernetics; San Diego, CA, USA; 2014. [Google Scholar]
49. Zhong X., Fu H., Yang J., Wang W. 8th IEEE Int Symp Dependable, Auton Secur Comput DASC 2009. 2009. Automatic segmentation in tongue image by mouth location and active appearance model; pp. 413–417. [Google Scholar]
50. Ning J., Zhang D., Wu C., Yue F. Automatic tongue image segmentation based on gradient vector flow and region merging. Neural Comput Appl. 2012;21:1819–1826. [Google Scholar]
51. Zhu M., Du J., Ding C. A comparative study of contemporary color tongue image extraction methods based on HSI. Int J Biomed Imaging. 2014;2014 [PMC free article] [PubMed] [Google Scholar]
52. Zhu M.F., Du J.Q., Zhang K., Ding C.H. A novel approach for tongue image extraction based on combination of color and space information. 2009 3rd International Conference on Bioinformatics and Biomedical Engineering; Beijing; 2009. pp. 1–4. [Google Scholar]
53. Liang C., Shi D. Proceedings – 2012 International Conference on Computer Science and Electronics Engineering, ICCSEE 2012, vol 2. Hangzhou. 2012. A prior knowledge-based algorithm for tongue body segmentation; pp. 646–649. [Google Scholar]
54. Hsu Y., Chen Y., Lo L., Chiang J.Y., Calibration A.C. Automatic tongue feature extraction. 2010 International Comput Symp, ICS2010; Tainan; 2010. pp. 936–941. [Google Scholar]
55. Jian-Qiang D., Yan-Sheng L., Ming-Feng Z., Kang Z., Cheng-Hua D. BioMedical Engineering and Informatics: New Development and the Future – Proceedings of the 1st International Conference on BioMedical Engineering and Informatics, BMEI 2008, vol. 1. Sanya. 2008. A novel algorithm of color tongue image segmentation based on HSI; pp. 733–737. [Google Scholar]
56. Li W., Hu S., Wang S., Xu S. Towards the objectification of tongue diagnosis: Automatic segmentation of tongue image. Industrial Electronics, 2009. IECON’09. 35th Annual Conference of IEEE; Porto; 2009. pp. 2121–2124. [Google Scholar]
57. Chen L., Wang B., Zhang Z., Lin F., Ma Y. Research on techniques of multifeatures extraction for tongue image and its application in retrieval. Comput Math Methods Med. 2017;2017:8064743. [PMC free article] [PubMed] [Google Scholar]
58. Kim S., Choi W., Yeo I., Nam D. Comparative analysis of tongue indices between patients with and without a self-reported yin deficiency: a cross-sectional study. Evid-based Complem Altern Med. 2017;2017:1279052. [PMC free article] [PubMed] [Google Scholar]
59. Kawanabe T., Kamarudin N.D., Ooi C.Y., Kobayashi F., Mi X., Sekine M. Quantification of tongue colour using machine learning in Kampo medicine. Eur J Integr Med. 2016 [Google Scholar]
60. Pang B., Zhang D., Wang K. Tongue image analysis for appendicitis diagnosis. Int J Sci Eng Technol Res. 2015;4:2263–2268. [Google Scholar]
61. Chen L., Wang D., Liu Y., Gao X., Shang H. A novel automatic tongue image segmentation algorithm: color enhancement method based on L*a*b* color space. 2015 IEEE International Conference on Bioinformatics and Biomedicine (BIBM); Washington, DC: IEEE; 2015. pp. 990–993. [Google Scholar]
62. Geng X. Label Distribution Learning. IEEE Trans Knowl Data Eng. 2014;4347:1–14. [Google Scholar]
63. Jung C., Kim K., Ku B., Kim J. Trends in tongue color of subtype patterns on deficiency syndrome. Integr Med Res. 2015;4:29. [Google Scholar]
64. Amato F., López A., Peña-Méndez E.M., Vaňhara P., Hampl A., Havel J. Artificial neural networks in medical diagnosis. J Appl Biomed. 2013;11:47–58. [Google Scholar]
65. Barbosa D.C., Roupar D.B., Ramos J.C., Tavares A.C., Lima C.S. Automatic small bowel tumor diagnosis by using multi-scale wavelet-based analysis in wireless capsule endoscopy images. Biomed Eng Online. 2012;11:3. [PMC free article] [PubMed] [Google Scholar]
66. Li J., Xu B., Ban X., Tai P., Ma B. A tongue image segmentation method based on enhanced HSV convolutional neural network. In: Luo Y., editor. Cooperative Design, Visualization, and Engineering: 14th International Conference, CDVE 2017. Mallorca, Spain, September 17–20, 2017, Proceedings. Springer International Publishing; Cham: 2017. pp. 252–260. [Google Scholar]
67. Cheung V., Westland S., Connah D., Ripamonti C. A comparative study of the characterisation of colour cameras by means of neural networks and polynomial transforms. Color Technol. 2004;120:19–25. [Google Scholar]
68. Zhuo L., Zhang P., Qu P., Peng Y., Zhang J., Li X. A K-PLSR-based color correction method for TCM tongue images under different illumination conditions. Neurocomputing. 2016;174:815–821. [Google Scholar]
69. Zhu M., Du J., Zhang K., He Y., Ding C. Research of tongue color recognition based on BP-ANN with self-adaptive network structure. Proceedings – 2015 7th International Conference on Information Technology in Medicine and Education, ITME 2015; Huangshan; 2016. pp. 208–211. [Google Scholar]
70. Gui M., Zhang X., Hu G., Zhang C., Zhang Z. 8th Int Conf Biomed Eng Informatics (BMEI 2015) 2015. A study on tongue image color description based on label distribution learning; pp. 148–152. [Google Scholar]
71. Deng Y., Manjunath B.S. Unsupervised segmentation of color-texture regions in images and video. IEEE Trans Pattern Anal Mach Intell. 2001;23:800–810. [Google Scholar]
72. Deng Y.D.Y., Kenney C., Moore M.S., Manjunath B.S. ISCAS’99 Proc 1999 IEEE Int Symp Circuits Syst VLSI (Cat. No. 99CH36349), vol. 4. 1999. Peer group filtering and perceptual color image quantization; pp. 21–24. [Google Scholar]
73. Canny J. A computational approach to edge detection. IEEE Trans Pattern Anal Mach Intell. 1986;8:679–698. [PubMed] [Google Scholar]
74. Kanawong R., Xu D. 2011 IEEE 13th International Conference on E-Health Networking, Applications and Services. IEEE. 2011. An automatic tongue detection and segmentation framework for computer-aided tongue image analysis; pp. 189–192. [Google Scholar]
75. Shi M.J., Li G.Z., Li F.F. C2G2FSnake: automatic tongue image segmentation utilizing prior knowledge. Sci China Inf Sci. 2013;56:1–14. [Google Scholar]
76. Xu W., Kanawong R., Xu D., Li S., Ma T., Zhang G. An automatic tongue detection and segmentation framework for computer-aided tongue image analysis. 2011 IEEE 13th International Conference on E-Health Networking, Applications and Services, HEALTHCOM 2011; Columbia, MO: IEEE; 2011. pp. 189–192. [Google Scholar]
77. Kass M., Witkin A., Terzopoulos D. Snakes: active contour models. Int J Comput Vis. 1988;1:321–331. [Google Scholar]
78. Zhai X.M., Lu H.D., Zhang L.Z. Application of image segmentation technique in tongue diagnosis. Proceedings – 2009 International Forum on Information Technology and Applications, IFITA 2009, vol. 2; Chengdu; 2009. pp. 768–771. [Google Scholar]
79. Shi M., Li G.Z., Li F., Xu C. A novel tongue segmentation approach utilizing double geodesic flow. ICCSE 2012 – Proceedings of 2012 7th International Conference on Computer Science and Education; Melbourne, VIC; 2012. pp. 21–25. [Google Scholar]
80. Faundra M.R., Ratna D., Yang X., Liu C., Ringenberg J., Deo M. IOP Conference Series: Materials Science and Engineering, vol. 755. 2016. Tongue segmentation using active contour model; p. 11001. [Google Scholar]
81. Wu J., Zhang Y., Bai J. Tongue area extraction in tongue diagnosis of traditional chinese medicine. Conference Proceedings: Annual International Conference of the IEEE Engineering in Medicine and Biology Society. IEEE Engineering in Medicine and Biology Society Conference, vol. 5; Shanghai; 2005. pp. 4955–4957. [PubMed] [Google Scholar]
82. Eddins S. 2006. Cell segmentation. MathWorks. Website: http://blogs.mathworks.com/steve/2006/06/02/cell-segmentation [accessed 08.02.17] [Google Scholar]
83. Madkour a., Hossain M.a., Dahal K.P., Yu H. Intelligent learning algorithms for active vibration control. IEEE Trans Syst Man Cybern C: Appl Rev. 2007;37:1022–1033. [Google Scholar]
84. Xu J., Xu Z., Lu P., Guo R., Yan H., Xu W. Classifying syndromes in Chinese medicine using multi-label learning algorithm with relevant features for each label. Chin J Integr Med. 2016;22:867–871. [PubMed] [Google Scholar]
85. Zhao Y., He L., Liu B., Li J., Li F., Huo R. Syndrome classification based on manifold ranking for viral hepatitis. Chin J Integr Med. 2014;20:394–399. [PubMed] [Google Scholar]
86. Huang Y., Sun M., Hsu P., Chen Y., Chiang J.Y., Lo L. The relationship between ischemic stroke patients with and without retroflex tongue: a retrospective study. Evid-based Complem Altern Med. 2017;2017 [PMC free article] [PubMed] [Google Scholar]
87. Kim J., Kim H., Kim K.H. Effects of Bu-Zhong-Yi-Qi-Tang for the treatment of functional dyspepsia: a feasibility study protocol. Integr Med Res. 2017;6:317–324. [PMC free article] [PubMed] [Google Scholar]
88. Halldorsson B.V., Bjornsson A.H., Gudmundsson H.T., Birgisson E.O., Ludviksson B.R., Gudbjornsson B. A clinical decision support system for the diagnosis, fracture risks and treatment of osteoporosis. Comput Math Methods Med. 2015;2015 [PMC free article] [PubMed] [Google Scholar]
89. Kunjunninair A.P. Clinical decision support system: risk level prediction of heart disease using weighted fuzzy rules and decision tree rules. Cent Eur J Comput Sci. 2012;2 86-86. [Google Scholar]
90. Abe S., Ishihara K., Adachi M., Okuda K. Tongue-coating as risk indicator for aspiration pneumonia in edentate elderly. Arch Gerontol Geriatr. 2008;47:267–275. [PubMed] [Google Scholar]
91. Zhang J., Xu J., Hu X., Chen Q., Tu L., Huang J. Diagnostic method of diabetes based on support vector machine and tongue images. Biomed Res Int. 2017;2017:1–9. [PMC free article] [PubMed] [Google Scholar]
92. Meng D., Cao G., Duan Y., Zhu M., Tu L., Xu D. Tongue images classification based on constrained high dispersal network. Evid-based Complem Altern Med. 2017;2017 [PMC free article] [PubMed] [Google Scholar]
93. Lo L., chien, Cheng T.L., Chen Y.J., Natsagdorj S., Chiang J.Y. TCM tongue diagnosis index of early-stage breast cancer. Complem Ther Med. 2015;23:705–713. [PubMed] [Google Scholar]
94. Gabhale B., Shinde M., Kamble A., Kulloli M. Tongue image analysis with color and gist features for diabetes diagnosis. Int Res J Eng Technol. 2017;4:523–526. [Google Scholar]
95. Bourouis A., Feham M., Hossain M.A., Zhang L. An intelligent mobile based decision support system for retinal disease diagnosis. Decis Support Syst. 2014;59:341–350. [Google Scholar]
96. Xu D. 2015. iTongue. iTunes. Website: https://itunes.apple.com/gb/app/itongue/id998044356?mt=8 [accessed 30.12.16] [Google Scholar]
97. Otsu N. A threshold selection method from gray-level histograms. IEEE Trans Syst Man Cybern. 1979;9:62–66. [Google Scholar]
98. Ini R. TongueDx: a tongue diagnosis system for personal health care on smartphones. Proceedings of the 5th Augmented Human International Conference, AH’14; New York; 2014. pp. 405–407. [Google Scholar]
99. Xu Y., Yang J.-Y., Jin Z. A novel method for fisher discriminant analysis. Pattern Recognit. 2004;37:381–384. [Google Scholar]